getItem with argument is column name
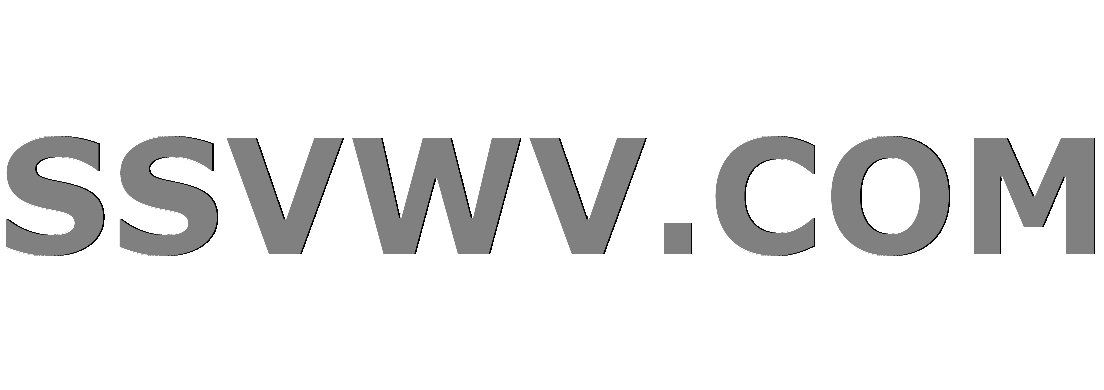
Multi tool use
up vote
0
down vote
favorite
My column col1
is an Array.
I know that col1.getItem(2)
allows you to access the second argument of the column. Is there a function to access with argument as column col1.getItem(col2)
?
I can create a UDF but I would have to specify which type the array is (and it can be multiple type) so a generic way would be better and welcome !
The UDF I use:
def retrieveByIndexSingle[T : ClassTag](value:Seq[T] ,index:Int,offset:Int=0):T = value(index + offset)
def retrieveByIndexSingleDUDF = udf((value:Seq[Double] ,index:Int) => {
retrieveByIndexSingle[Double](value, index)
})
def retrieveByIndexSingleSUDF = udf((value:Seq[String] ,index:Int) => {
retrieveByIndexSingle[String](value, index)
})
scala apache-spark user-defined-functions
add a comment |
up vote
0
down vote
favorite
My column col1
is an Array.
I know that col1.getItem(2)
allows you to access the second argument of the column. Is there a function to access with argument as column col1.getItem(col2)
?
I can create a UDF but I would have to specify which type the array is (and it can be multiple type) so a generic way would be better and welcome !
The UDF I use:
def retrieveByIndexSingle[T : ClassTag](value:Seq[T] ,index:Int,offset:Int=0):T = value(index + offset)
def retrieveByIndexSingleDUDF = udf((value:Seq[Double] ,index:Int) => {
retrieveByIndexSingle[Double](value, index)
})
def retrieveByIndexSingleSUDF = udf((value:Seq[String] ,index:Int) => {
retrieveByIndexSingle[String](value, index)
})
scala apache-spark user-defined-functions
add a comment |
up vote
0
down vote
favorite
up vote
0
down vote
favorite
My column col1
is an Array.
I know that col1.getItem(2)
allows you to access the second argument of the column. Is there a function to access with argument as column col1.getItem(col2)
?
I can create a UDF but I would have to specify which type the array is (and it can be multiple type) so a generic way would be better and welcome !
The UDF I use:
def retrieveByIndexSingle[T : ClassTag](value:Seq[T] ,index:Int,offset:Int=0):T = value(index + offset)
def retrieveByIndexSingleDUDF = udf((value:Seq[Double] ,index:Int) => {
retrieveByIndexSingle[Double](value, index)
})
def retrieveByIndexSingleSUDF = udf((value:Seq[String] ,index:Int) => {
retrieveByIndexSingle[String](value, index)
})
scala apache-spark user-defined-functions
My column col1
is an Array.
I know that col1.getItem(2)
allows you to access the second argument of the column. Is there a function to access with argument as column col1.getItem(col2)
?
I can create a UDF but I would have to specify which type the array is (and it can be multiple type) so a generic way would be better and welcome !
The UDF I use:
def retrieveByIndexSingle[T : ClassTag](value:Seq[T] ,index:Int,offset:Int=0):T = value(index + offset)
def retrieveByIndexSingleDUDF = udf((value:Seq[Double] ,index:Int) => {
retrieveByIndexSingle[Double](value, index)
})
def retrieveByIndexSingleSUDF = udf((value:Seq[String] ,index:Int) => {
retrieveByIndexSingle[String](value, index)
})
scala apache-spark user-defined-functions
scala apache-spark user-defined-functions
asked Nov 19 at 23:21


Guillaume G
751714
751714
add a comment |
add a comment |
1 Answer
1
active
oldest
votes
up vote
1
down vote
accepted
It is possible to use SQL expression for example with expr
:
import org.apache.spark.sql.functions.expr
val df = Seq(
(Seq("a", "b", "c"), 0), (Seq("d", "e", "f"), 2)
).toDF("col1", "col2")
df.withColumn("col3", expr("col1[col2]")).show
+---------+----+----+
| col1|col2|col3|
+---------+----+----+
|[a, b, c]| 0| a|
|[d, e, f]| 2| f|
+---------+----+----+
or, in Spark 2.4 or later, element_at
function:
import org.apache.spark.sql.functions.element_at
df.withColumn("col3", element_at($"col1", $"col2" + 1)).show
+---------+----+----+
| col1|col2|col3|
+---------+----+----+
|[a, b, c]| 0| a|
|[d, e, f]| 2| f|
+---------+----+----+
Please note that at the moment (Spark 2.4) there is inconsistency between these two methods:
- SQL
indexing is 0-based.
element_at
indexing is 1-based.
thanks. The 1-based notation is terrible ...
– Guillaume G
Nov 20 at 2:46
add a comment |
1 Answer
1
active
oldest
votes
1 Answer
1
active
oldest
votes
active
oldest
votes
active
oldest
votes
up vote
1
down vote
accepted
It is possible to use SQL expression for example with expr
:
import org.apache.spark.sql.functions.expr
val df = Seq(
(Seq("a", "b", "c"), 0), (Seq("d", "e", "f"), 2)
).toDF("col1", "col2")
df.withColumn("col3", expr("col1[col2]")).show
+---------+----+----+
| col1|col2|col3|
+---------+----+----+
|[a, b, c]| 0| a|
|[d, e, f]| 2| f|
+---------+----+----+
or, in Spark 2.4 or later, element_at
function:
import org.apache.spark.sql.functions.element_at
df.withColumn("col3", element_at($"col1", $"col2" + 1)).show
+---------+----+----+
| col1|col2|col3|
+---------+----+----+
|[a, b, c]| 0| a|
|[d, e, f]| 2| f|
+---------+----+----+
Please note that at the moment (Spark 2.4) there is inconsistency between these two methods:
- SQL
indexing is 0-based.
element_at
indexing is 1-based.
thanks. The 1-based notation is terrible ...
– Guillaume G
Nov 20 at 2:46
add a comment |
up vote
1
down vote
accepted
It is possible to use SQL expression for example with expr
:
import org.apache.spark.sql.functions.expr
val df = Seq(
(Seq("a", "b", "c"), 0), (Seq("d", "e", "f"), 2)
).toDF("col1", "col2")
df.withColumn("col3", expr("col1[col2]")).show
+---------+----+----+
| col1|col2|col3|
+---------+----+----+
|[a, b, c]| 0| a|
|[d, e, f]| 2| f|
+---------+----+----+
or, in Spark 2.4 or later, element_at
function:
import org.apache.spark.sql.functions.element_at
df.withColumn("col3", element_at($"col1", $"col2" + 1)).show
+---------+----+----+
| col1|col2|col3|
+---------+----+----+
|[a, b, c]| 0| a|
|[d, e, f]| 2| f|
+---------+----+----+
Please note that at the moment (Spark 2.4) there is inconsistency between these two methods:
- SQL
indexing is 0-based.
element_at
indexing is 1-based.
thanks. The 1-based notation is terrible ...
– Guillaume G
Nov 20 at 2:46
add a comment |
up vote
1
down vote
accepted
up vote
1
down vote
accepted
It is possible to use SQL expression for example with expr
:
import org.apache.spark.sql.functions.expr
val df = Seq(
(Seq("a", "b", "c"), 0), (Seq("d", "e", "f"), 2)
).toDF("col1", "col2")
df.withColumn("col3", expr("col1[col2]")).show
+---------+----+----+
| col1|col2|col3|
+---------+----+----+
|[a, b, c]| 0| a|
|[d, e, f]| 2| f|
+---------+----+----+
or, in Spark 2.4 or later, element_at
function:
import org.apache.spark.sql.functions.element_at
df.withColumn("col3", element_at($"col1", $"col2" + 1)).show
+---------+----+----+
| col1|col2|col3|
+---------+----+----+
|[a, b, c]| 0| a|
|[d, e, f]| 2| f|
+---------+----+----+
Please note that at the moment (Spark 2.4) there is inconsistency between these two methods:
- SQL
indexing is 0-based.
element_at
indexing is 1-based.
It is possible to use SQL expression for example with expr
:
import org.apache.spark.sql.functions.expr
val df = Seq(
(Seq("a", "b", "c"), 0), (Seq("d", "e", "f"), 2)
).toDF("col1", "col2")
df.withColumn("col3", expr("col1[col2]")).show
+---------+----+----+
| col1|col2|col3|
+---------+----+----+
|[a, b, c]| 0| a|
|[d, e, f]| 2| f|
+---------+----+----+
or, in Spark 2.4 or later, element_at
function:
import org.apache.spark.sql.functions.element_at
df.withColumn("col3", element_at($"col1", $"col2" + 1)).show
+---------+----+----+
| col1|col2|col3|
+---------+----+----+
|[a, b, c]| 0| a|
|[d, e, f]| 2| f|
+---------+----+----+
Please note that at the moment (Spark 2.4) there is inconsistency between these two methods:
- SQL
indexing is 0-based.
element_at
indexing is 1-based.
edited Nov 20 at 1:10
answered Nov 20 at 1:05
user10465355
1,164310
1,164310
thanks. The 1-based notation is terrible ...
– Guillaume G
Nov 20 at 2:46
add a comment |
thanks. The 1-based notation is terrible ...
– Guillaume G
Nov 20 at 2:46
thanks. The 1-based notation is terrible ...
– Guillaume G
Nov 20 at 2:46
thanks. The 1-based notation is terrible ...
– Guillaume G
Nov 20 at 2:46
add a comment |
Thanks for contributing an answer to Stack Overflow!
- Please be sure to answer the question. Provide details and share your research!
But avoid …
- Asking for help, clarification, or responding to other answers.
- Making statements based on opinion; back them up with references or personal experience.
To learn more, see our tips on writing great answers.
Some of your past answers have not been well-received, and you're in danger of being blocked from answering.
Please pay close attention to the following guidance:
- Please be sure to answer the question. Provide details and share your research!
But avoid …
- Asking for help, clarification, or responding to other answers.
- Making statements based on opinion; back them up with references or personal experience.
To learn more, see our tips on writing great answers.
Sign up or log in
StackExchange.ready(function () {
StackExchange.helpers.onClickDraftSave('#login-link');
});
Sign up using Google
Sign up using Facebook
Sign up using Email and Password
Post as a guest
Required, but never shown
StackExchange.ready(
function () {
StackExchange.openid.initPostLogin('.new-post-login', 'https%3a%2f%2fstackoverflow.com%2fquestions%2f53384054%2fgetitem-with-argument-is-column-name%23new-answer', 'question_page');
}
);
Post as a guest
Required, but never shown
Sign up or log in
StackExchange.ready(function () {
StackExchange.helpers.onClickDraftSave('#login-link');
});
Sign up using Google
Sign up using Facebook
Sign up using Email and Password
Post as a guest
Required, but never shown
Sign up or log in
StackExchange.ready(function () {
StackExchange.helpers.onClickDraftSave('#login-link');
});
Sign up using Google
Sign up using Facebook
Sign up using Email and Password
Post as a guest
Required, but never shown
Sign up or log in
StackExchange.ready(function () {
StackExchange.helpers.onClickDraftSave('#login-link');
});
Sign up using Google
Sign up using Facebook
Sign up using Email and Password
Sign up using Google
Sign up using Facebook
Sign up using Email and Password
Post as a guest
Required, but never shown
Required, but never shown
Required, but never shown
Required, but never shown
Required, but never shown
Required, but never shown
Required, but never shown
Required, but never shown
Required, but never shown
RZwhwSf63,KaAimzHTHeIKaS,AwwJRi