find most visited node in a graph
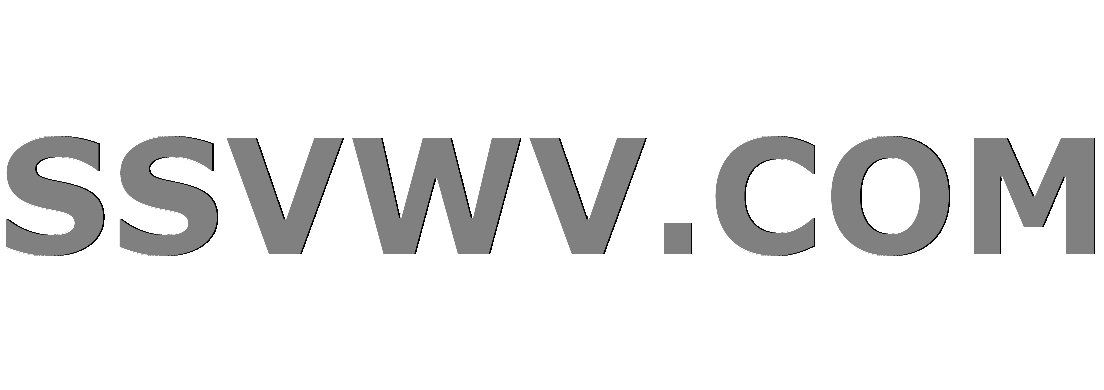
Multi tool use
There are N nodes in a graph connected by exactly N-1 edges. There is exactly 1 shortest path from one node to any other node. The nodes are numbered from 1 to N. Given Q queries which tell source node and the destination nodes. Find the most visited node after traveling those Q paths. For example, say Q=3
and 3 queries are :
1 5
2 4
3 1
So travel from node 1 to node 5, then from node 2 to node 4, then from node 3 to node 1. Finally, find what is the most visited node after the Q queries.
Finding every path and incrementing every visited node count is a naive approach. The interviewer asked me to optimize it.
graph tree least-common-ancestor
add a comment |
There are N nodes in a graph connected by exactly N-1 edges. There is exactly 1 shortest path from one node to any other node. The nodes are numbered from 1 to N. Given Q queries which tell source node and the destination nodes. Find the most visited node after traveling those Q paths. For example, say Q=3
and 3 queries are :
1 5
2 4
3 1
So travel from node 1 to node 5, then from node 2 to node 4, then from node 3 to node 1. Finally, find what is the most visited node after the Q queries.
Finding every path and incrementing every visited node count is a naive approach. The interviewer asked me to optimize it.
graph tree least-common-ancestor
Are you already given these Q paths or it is intention to inquire as less paths as possible to deduce which node is most visited? Result is one node or all nodes with same number of visits? I suppose that end nodes are also counted as visited?
– Ante
Nov 24 '18 at 7:32
Only source and destination nodes are given, not the paths. After travelling all those source to destination paths, some nodes will have max visited count. We need to find that count.
– zuccy
Nov 24 '18 at 7:57
add a comment |
There are N nodes in a graph connected by exactly N-1 edges. There is exactly 1 shortest path from one node to any other node. The nodes are numbered from 1 to N. Given Q queries which tell source node and the destination nodes. Find the most visited node after traveling those Q paths. For example, say Q=3
and 3 queries are :
1 5
2 4
3 1
So travel from node 1 to node 5, then from node 2 to node 4, then from node 3 to node 1. Finally, find what is the most visited node after the Q queries.
Finding every path and incrementing every visited node count is a naive approach. The interviewer asked me to optimize it.
graph tree least-common-ancestor
There are N nodes in a graph connected by exactly N-1 edges. There is exactly 1 shortest path from one node to any other node. The nodes are numbered from 1 to N. Given Q queries which tell source node and the destination nodes. Find the most visited node after traveling those Q paths. For example, say Q=3
and 3 queries are :
1 5
2 4
3 1
So travel from node 1 to node 5, then from node 2 to node 4, then from node 3 to node 1. Finally, find what is the most visited node after the Q queries.
Finding every path and incrementing every visited node count is a naive approach. The interviewer asked me to optimize it.
graph tree least-common-ancestor
graph tree least-common-ancestor
edited Nov 24 '18 at 0:00


Matias Barrios
1,542316
1,542316
asked Nov 23 '18 at 16:44
zuccyzuccy
91
91
Are you already given these Q paths or it is intention to inquire as less paths as possible to deduce which node is most visited? Result is one node or all nodes with same number of visits? I suppose that end nodes are also counted as visited?
– Ante
Nov 24 '18 at 7:32
Only source and destination nodes are given, not the paths. After travelling all those source to destination paths, some nodes will have max visited count. We need to find that count.
– zuccy
Nov 24 '18 at 7:57
add a comment |
Are you already given these Q paths or it is intention to inquire as less paths as possible to deduce which node is most visited? Result is one node or all nodes with same number of visits? I suppose that end nodes are also counted as visited?
– Ante
Nov 24 '18 at 7:32
Only source and destination nodes are given, not the paths. After travelling all those source to destination paths, some nodes will have max visited count. We need to find that count.
– zuccy
Nov 24 '18 at 7:57
Are you already given these Q paths or it is intention to inquire as less paths as possible to deduce which node is most visited? Result is one node or all nodes with same number of visits? I suppose that end nodes are also counted as visited?
– Ante
Nov 24 '18 at 7:32
Are you already given these Q paths or it is intention to inquire as less paths as possible to deduce which node is most visited? Result is one node or all nodes with same number of visits? I suppose that end nodes are also counted as visited?
– Ante
Nov 24 '18 at 7:32
Only source and destination nodes are given, not the paths. After travelling all those source to destination paths, some nodes will have max visited count. We need to find that count.
– zuccy
Nov 24 '18 at 7:57
Only source and destination nodes are given, not the paths. After travelling all those source to destination paths, some nodes will have max visited count. We need to find that count.
– zuccy
Nov 24 '18 at 7:57
add a comment |
1 Answer
1
active
oldest
votes
Optimization often involves tradeoffs; in some cases one algorithm is unambiguously better than another, but in other cases one algorithm is better in one respect (e.g. time) and a different algorithm is better in a different respect (e.g. memory usage).
In your case, I'm guessing that your interviewer was looking for an approach that optimizes for the amount of processing that has to be done after you start receiving queries, even if this means you have to do more preprocessing on the graph. My reason for saying this is the term "query"; it's quite common to optimize a data source for "online" querying. (Of course, (s)he probably didn't expect you to decide on your own that this tradeoff was OK; rather, (s)he was likely hoping for a conversation about the different sorts of tradeoffs.)
So, with that in mind . . .
- I see that you've already tagged your question with [tree] and [least-common-answer], so you've presumably already made the biggest observations, namely:
- The graph is a tree. We can arbitrarily select a "root", such that every other node has a "parent", a nonzero "depth", one or more "ancestors", etc.
- Once we've done that, the shortest path from node a to node b consists of node a, node b, all ancestors of a that aren't ancestors of b, all ancestors of b that aren't ancestors of a, and their "least common ancestor". (This remains true if a is an ancestor of b or vice versa: if a is an ancestor of b, then it's the least common ancestor of a and b, and vice versa. It even remains true if a and b are the same.)
- So, we can do the following preprocessing:
- Represent the graph as a mapping from each node to a list of its neighbors. (Since the nodes are numbered from 1 to N, this mapping is an array of N lists.)
- Choose a root node.
- Calculate and store each node's "parent" and "depth". (We can do this in O(N) time using depth-first search or breadth-first search.)
- For each pair of nodes, calculate and store their "least common ancestor". (We can do this in total time O(N2) using the results of the previous step and memoization, because the memoization provides amortization.)
- Initialize a mapping from each node to the number of times that it's the endpoint of a path, and a mapping from each node to the number of times that it's the least common ancestor of the endpoints of a path. (Note: if a given path is from a single node to itself, then we will count that as twice that it's the endpoint of a path — as well as once that it's the last common ancestor of the endpoints.)
- For each query, update the two mappings. We can do this in O(1) time per query, for a total of O(Q) time.
- Finally:
- Do a post-order traversal of the graph, computing the number of paths that visited that node. The logic for this is as follows: the total number of paths that visited node a is equal to the sum of the numbers of paths that visited each of its children, minus the sum of the numbers of times that each of its children was the last common ancestor of a path's endpoint, plus the number of times that a itself was an endpoint, minus the number of times that a itself was the last common ancestor of a path's endpoint (to cancel out double-counting).
- Return the node for which the previous step returned the greatest number. If multiple nodes are tied for greatest, then . . . I dunno, the problem statement was vague about this, you'll need to ask for requirements.
Overall, this requires O(N2) preprocessing, O(Q) realtime processing per query, and O(N) postprocessing.
If N is quite large, and we expect it to be possible that only a small subset of nodes were visited even once, then we can speed up the postprocessing by ignoring unvisited parts of the tree. This involves maintaining a set of nodes that were endpoints of paths, and then doing the postprocessing in "bottom-up" fashion, starting at the deepest such nodes, and moving "parentward" from a given node only if the number of paths that visited that node is less than the number of times it was a lest common ancestor. If we denote the number of distinct endpoints by P and the number of distinct visited nodes by M, then this can be done in something like O(P log P + M).
Thanks for your answer. I was thinking there might be a simple logic between the nodes and the LCA of nodes. Because the interviewer asked me to find the relationship between LCA and nodes. BTW N and Q values are upto 100000.
– zuccy
Nov 26 '18 at 5:08
@zuccy: Did the interviewer say anything about how the graph is represented?
– ruakh
Nov 26 '18 at 5:56
Thank you senpai.
– zuccy
Nov 26 '18 at 18:10
add a comment |
Your Answer
StackExchange.ifUsing("editor", function () {
StackExchange.using("externalEditor", function () {
StackExchange.using("snippets", function () {
StackExchange.snippets.init();
});
});
}, "code-snippets");
StackExchange.ready(function() {
var channelOptions = {
tags: "".split(" "),
id: "1"
};
initTagRenderer("".split(" "), "".split(" "), channelOptions);
StackExchange.using("externalEditor", function() {
// Have to fire editor after snippets, if snippets enabled
if (StackExchange.settings.snippets.snippetsEnabled) {
StackExchange.using("snippets", function() {
createEditor();
});
}
else {
createEditor();
}
});
function createEditor() {
StackExchange.prepareEditor({
heartbeatType: 'answer',
autoActivateHeartbeat: false,
convertImagesToLinks: true,
noModals: true,
showLowRepImageUploadWarning: true,
reputationToPostImages: 10,
bindNavPrevention: true,
postfix: "",
imageUploader: {
brandingHtml: "Powered by u003ca class="icon-imgur-white" href="https://imgur.com/"u003eu003c/au003e",
contentPolicyHtml: "User contributions licensed under u003ca href="https://creativecommons.org/licenses/by-sa/3.0/"u003ecc by-sa 3.0 with attribution requiredu003c/au003e u003ca href="https://stackoverflow.com/legal/content-policy"u003e(content policy)u003c/au003e",
allowUrls: true
},
onDemand: true,
discardSelector: ".discard-answer"
,immediatelyShowMarkdownHelp:true
});
}
});
Sign up or log in
StackExchange.ready(function () {
StackExchange.helpers.onClickDraftSave('#login-link');
});
Sign up using Google
Sign up using Facebook
Sign up using Email and Password
Post as a guest
Required, but never shown
StackExchange.ready(
function () {
StackExchange.openid.initPostLogin('.new-post-login', 'https%3a%2f%2fstackoverflow.com%2fquestions%2f53450334%2ffind-most-visited-node-in-a-graph%23new-answer', 'question_page');
}
);
Post as a guest
Required, but never shown
1 Answer
1
active
oldest
votes
1 Answer
1
active
oldest
votes
active
oldest
votes
active
oldest
votes
Optimization often involves tradeoffs; in some cases one algorithm is unambiguously better than another, but in other cases one algorithm is better in one respect (e.g. time) and a different algorithm is better in a different respect (e.g. memory usage).
In your case, I'm guessing that your interviewer was looking for an approach that optimizes for the amount of processing that has to be done after you start receiving queries, even if this means you have to do more preprocessing on the graph. My reason for saying this is the term "query"; it's quite common to optimize a data source for "online" querying. (Of course, (s)he probably didn't expect you to decide on your own that this tradeoff was OK; rather, (s)he was likely hoping for a conversation about the different sorts of tradeoffs.)
So, with that in mind . . .
- I see that you've already tagged your question with [tree] and [least-common-answer], so you've presumably already made the biggest observations, namely:
- The graph is a tree. We can arbitrarily select a "root", such that every other node has a "parent", a nonzero "depth", one or more "ancestors", etc.
- Once we've done that, the shortest path from node a to node b consists of node a, node b, all ancestors of a that aren't ancestors of b, all ancestors of b that aren't ancestors of a, and their "least common ancestor". (This remains true if a is an ancestor of b or vice versa: if a is an ancestor of b, then it's the least common ancestor of a and b, and vice versa. It even remains true if a and b are the same.)
- So, we can do the following preprocessing:
- Represent the graph as a mapping from each node to a list of its neighbors. (Since the nodes are numbered from 1 to N, this mapping is an array of N lists.)
- Choose a root node.
- Calculate and store each node's "parent" and "depth". (We can do this in O(N) time using depth-first search or breadth-first search.)
- For each pair of nodes, calculate and store their "least common ancestor". (We can do this in total time O(N2) using the results of the previous step and memoization, because the memoization provides amortization.)
- Initialize a mapping from each node to the number of times that it's the endpoint of a path, and a mapping from each node to the number of times that it's the least common ancestor of the endpoints of a path. (Note: if a given path is from a single node to itself, then we will count that as twice that it's the endpoint of a path — as well as once that it's the last common ancestor of the endpoints.)
- For each query, update the two mappings. We can do this in O(1) time per query, for a total of O(Q) time.
- Finally:
- Do a post-order traversal of the graph, computing the number of paths that visited that node. The logic for this is as follows: the total number of paths that visited node a is equal to the sum of the numbers of paths that visited each of its children, minus the sum of the numbers of times that each of its children was the last common ancestor of a path's endpoint, plus the number of times that a itself was an endpoint, minus the number of times that a itself was the last common ancestor of a path's endpoint (to cancel out double-counting).
- Return the node for which the previous step returned the greatest number. If multiple nodes are tied for greatest, then . . . I dunno, the problem statement was vague about this, you'll need to ask for requirements.
Overall, this requires O(N2) preprocessing, O(Q) realtime processing per query, and O(N) postprocessing.
If N is quite large, and we expect it to be possible that only a small subset of nodes were visited even once, then we can speed up the postprocessing by ignoring unvisited parts of the tree. This involves maintaining a set of nodes that were endpoints of paths, and then doing the postprocessing in "bottom-up" fashion, starting at the deepest such nodes, and moving "parentward" from a given node only if the number of paths that visited that node is less than the number of times it was a lest common ancestor. If we denote the number of distinct endpoints by P and the number of distinct visited nodes by M, then this can be done in something like O(P log P + M).
Thanks for your answer. I was thinking there might be a simple logic between the nodes and the LCA of nodes. Because the interviewer asked me to find the relationship between LCA and nodes. BTW N and Q values are upto 100000.
– zuccy
Nov 26 '18 at 5:08
@zuccy: Did the interviewer say anything about how the graph is represented?
– ruakh
Nov 26 '18 at 5:56
Thank you senpai.
– zuccy
Nov 26 '18 at 18:10
add a comment |
Optimization often involves tradeoffs; in some cases one algorithm is unambiguously better than another, but in other cases one algorithm is better in one respect (e.g. time) and a different algorithm is better in a different respect (e.g. memory usage).
In your case, I'm guessing that your interviewer was looking for an approach that optimizes for the amount of processing that has to be done after you start receiving queries, even if this means you have to do more preprocessing on the graph. My reason for saying this is the term "query"; it's quite common to optimize a data source for "online" querying. (Of course, (s)he probably didn't expect you to decide on your own that this tradeoff was OK; rather, (s)he was likely hoping for a conversation about the different sorts of tradeoffs.)
So, with that in mind . . .
- I see that you've already tagged your question with [tree] and [least-common-answer], so you've presumably already made the biggest observations, namely:
- The graph is a tree. We can arbitrarily select a "root", such that every other node has a "parent", a nonzero "depth", one or more "ancestors", etc.
- Once we've done that, the shortest path from node a to node b consists of node a, node b, all ancestors of a that aren't ancestors of b, all ancestors of b that aren't ancestors of a, and their "least common ancestor". (This remains true if a is an ancestor of b or vice versa: if a is an ancestor of b, then it's the least common ancestor of a and b, and vice versa. It even remains true if a and b are the same.)
- So, we can do the following preprocessing:
- Represent the graph as a mapping from each node to a list of its neighbors. (Since the nodes are numbered from 1 to N, this mapping is an array of N lists.)
- Choose a root node.
- Calculate and store each node's "parent" and "depth". (We can do this in O(N) time using depth-first search or breadth-first search.)
- For each pair of nodes, calculate and store their "least common ancestor". (We can do this in total time O(N2) using the results of the previous step and memoization, because the memoization provides amortization.)
- Initialize a mapping from each node to the number of times that it's the endpoint of a path, and a mapping from each node to the number of times that it's the least common ancestor of the endpoints of a path. (Note: if a given path is from a single node to itself, then we will count that as twice that it's the endpoint of a path — as well as once that it's the last common ancestor of the endpoints.)
- For each query, update the two mappings. We can do this in O(1) time per query, for a total of O(Q) time.
- Finally:
- Do a post-order traversal of the graph, computing the number of paths that visited that node. The logic for this is as follows: the total number of paths that visited node a is equal to the sum of the numbers of paths that visited each of its children, minus the sum of the numbers of times that each of its children was the last common ancestor of a path's endpoint, plus the number of times that a itself was an endpoint, minus the number of times that a itself was the last common ancestor of a path's endpoint (to cancel out double-counting).
- Return the node for which the previous step returned the greatest number. If multiple nodes are tied for greatest, then . . . I dunno, the problem statement was vague about this, you'll need to ask for requirements.
Overall, this requires O(N2) preprocessing, O(Q) realtime processing per query, and O(N) postprocessing.
If N is quite large, and we expect it to be possible that only a small subset of nodes were visited even once, then we can speed up the postprocessing by ignoring unvisited parts of the tree. This involves maintaining a set of nodes that were endpoints of paths, and then doing the postprocessing in "bottom-up" fashion, starting at the deepest such nodes, and moving "parentward" from a given node only if the number of paths that visited that node is less than the number of times it was a lest common ancestor. If we denote the number of distinct endpoints by P and the number of distinct visited nodes by M, then this can be done in something like O(P log P + M).
Thanks for your answer. I was thinking there might be a simple logic between the nodes and the LCA of nodes. Because the interviewer asked me to find the relationship between LCA and nodes. BTW N and Q values are upto 100000.
– zuccy
Nov 26 '18 at 5:08
@zuccy: Did the interviewer say anything about how the graph is represented?
– ruakh
Nov 26 '18 at 5:56
Thank you senpai.
– zuccy
Nov 26 '18 at 18:10
add a comment |
Optimization often involves tradeoffs; in some cases one algorithm is unambiguously better than another, but in other cases one algorithm is better in one respect (e.g. time) and a different algorithm is better in a different respect (e.g. memory usage).
In your case, I'm guessing that your interviewer was looking for an approach that optimizes for the amount of processing that has to be done after you start receiving queries, even if this means you have to do more preprocessing on the graph. My reason for saying this is the term "query"; it's quite common to optimize a data source for "online" querying. (Of course, (s)he probably didn't expect you to decide on your own that this tradeoff was OK; rather, (s)he was likely hoping for a conversation about the different sorts of tradeoffs.)
So, with that in mind . . .
- I see that you've already tagged your question with [tree] and [least-common-answer], so you've presumably already made the biggest observations, namely:
- The graph is a tree. We can arbitrarily select a "root", such that every other node has a "parent", a nonzero "depth", one or more "ancestors", etc.
- Once we've done that, the shortest path from node a to node b consists of node a, node b, all ancestors of a that aren't ancestors of b, all ancestors of b that aren't ancestors of a, and their "least common ancestor". (This remains true if a is an ancestor of b or vice versa: if a is an ancestor of b, then it's the least common ancestor of a and b, and vice versa. It even remains true if a and b are the same.)
- So, we can do the following preprocessing:
- Represent the graph as a mapping from each node to a list of its neighbors. (Since the nodes are numbered from 1 to N, this mapping is an array of N lists.)
- Choose a root node.
- Calculate and store each node's "parent" and "depth". (We can do this in O(N) time using depth-first search or breadth-first search.)
- For each pair of nodes, calculate and store their "least common ancestor". (We can do this in total time O(N2) using the results of the previous step and memoization, because the memoization provides amortization.)
- Initialize a mapping from each node to the number of times that it's the endpoint of a path, and a mapping from each node to the number of times that it's the least common ancestor of the endpoints of a path. (Note: if a given path is from a single node to itself, then we will count that as twice that it's the endpoint of a path — as well as once that it's the last common ancestor of the endpoints.)
- For each query, update the two mappings. We can do this in O(1) time per query, for a total of O(Q) time.
- Finally:
- Do a post-order traversal of the graph, computing the number of paths that visited that node. The logic for this is as follows: the total number of paths that visited node a is equal to the sum of the numbers of paths that visited each of its children, minus the sum of the numbers of times that each of its children was the last common ancestor of a path's endpoint, plus the number of times that a itself was an endpoint, minus the number of times that a itself was the last common ancestor of a path's endpoint (to cancel out double-counting).
- Return the node for which the previous step returned the greatest number. If multiple nodes are tied for greatest, then . . . I dunno, the problem statement was vague about this, you'll need to ask for requirements.
Overall, this requires O(N2) preprocessing, O(Q) realtime processing per query, and O(N) postprocessing.
If N is quite large, and we expect it to be possible that only a small subset of nodes were visited even once, then we can speed up the postprocessing by ignoring unvisited parts of the tree. This involves maintaining a set of nodes that were endpoints of paths, and then doing the postprocessing in "bottom-up" fashion, starting at the deepest such nodes, and moving "parentward" from a given node only if the number of paths that visited that node is less than the number of times it was a lest common ancestor. If we denote the number of distinct endpoints by P and the number of distinct visited nodes by M, then this can be done in something like O(P log P + M).
Optimization often involves tradeoffs; in some cases one algorithm is unambiguously better than another, but in other cases one algorithm is better in one respect (e.g. time) and a different algorithm is better in a different respect (e.g. memory usage).
In your case, I'm guessing that your interviewer was looking for an approach that optimizes for the amount of processing that has to be done after you start receiving queries, even if this means you have to do more preprocessing on the graph. My reason for saying this is the term "query"; it's quite common to optimize a data source for "online" querying. (Of course, (s)he probably didn't expect you to decide on your own that this tradeoff was OK; rather, (s)he was likely hoping for a conversation about the different sorts of tradeoffs.)
So, with that in mind . . .
- I see that you've already tagged your question with [tree] and [least-common-answer], so you've presumably already made the biggest observations, namely:
- The graph is a tree. We can arbitrarily select a "root", such that every other node has a "parent", a nonzero "depth", one or more "ancestors", etc.
- Once we've done that, the shortest path from node a to node b consists of node a, node b, all ancestors of a that aren't ancestors of b, all ancestors of b that aren't ancestors of a, and their "least common ancestor". (This remains true if a is an ancestor of b or vice versa: if a is an ancestor of b, then it's the least common ancestor of a and b, and vice versa. It even remains true if a and b are the same.)
- So, we can do the following preprocessing:
- Represent the graph as a mapping from each node to a list of its neighbors. (Since the nodes are numbered from 1 to N, this mapping is an array of N lists.)
- Choose a root node.
- Calculate and store each node's "parent" and "depth". (We can do this in O(N) time using depth-first search or breadth-first search.)
- For each pair of nodes, calculate and store their "least common ancestor". (We can do this in total time O(N2) using the results of the previous step and memoization, because the memoization provides amortization.)
- Initialize a mapping from each node to the number of times that it's the endpoint of a path, and a mapping from each node to the number of times that it's the least common ancestor of the endpoints of a path. (Note: if a given path is from a single node to itself, then we will count that as twice that it's the endpoint of a path — as well as once that it's the last common ancestor of the endpoints.)
- For each query, update the two mappings. We can do this in O(1) time per query, for a total of O(Q) time.
- Finally:
- Do a post-order traversal of the graph, computing the number of paths that visited that node. The logic for this is as follows: the total number of paths that visited node a is equal to the sum of the numbers of paths that visited each of its children, minus the sum of the numbers of times that each of its children was the last common ancestor of a path's endpoint, plus the number of times that a itself was an endpoint, minus the number of times that a itself was the last common ancestor of a path's endpoint (to cancel out double-counting).
- Return the node for which the previous step returned the greatest number. If multiple nodes are tied for greatest, then . . . I dunno, the problem statement was vague about this, you'll need to ask for requirements.
Overall, this requires O(N2) preprocessing, O(Q) realtime processing per query, and O(N) postprocessing.
If N is quite large, and we expect it to be possible that only a small subset of nodes were visited even once, then we can speed up the postprocessing by ignoring unvisited parts of the tree. This involves maintaining a set of nodes that were endpoints of paths, and then doing the postprocessing in "bottom-up" fashion, starting at the deepest such nodes, and moving "parentward" from a given node only if the number of paths that visited that node is less than the number of times it was a lest common ancestor. If we denote the number of distinct endpoints by P and the number of distinct visited nodes by M, then this can be done in something like O(P log P + M).
answered Nov 25 '18 at 19:16
ruakhruakh
125k13201256
125k13201256
Thanks for your answer. I was thinking there might be a simple logic between the nodes and the LCA of nodes. Because the interviewer asked me to find the relationship between LCA and nodes. BTW N and Q values are upto 100000.
– zuccy
Nov 26 '18 at 5:08
@zuccy: Did the interviewer say anything about how the graph is represented?
– ruakh
Nov 26 '18 at 5:56
Thank you senpai.
– zuccy
Nov 26 '18 at 18:10
add a comment |
Thanks for your answer. I was thinking there might be a simple logic between the nodes and the LCA of nodes. Because the interviewer asked me to find the relationship between LCA and nodes. BTW N and Q values are upto 100000.
– zuccy
Nov 26 '18 at 5:08
@zuccy: Did the interviewer say anything about how the graph is represented?
– ruakh
Nov 26 '18 at 5:56
Thank you senpai.
– zuccy
Nov 26 '18 at 18:10
Thanks for your answer. I was thinking there might be a simple logic between the nodes and the LCA of nodes. Because the interviewer asked me to find the relationship between LCA and nodes. BTW N and Q values are upto 100000.
– zuccy
Nov 26 '18 at 5:08
Thanks for your answer. I was thinking there might be a simple logic between the nodes and the LCA of nodes. Because the interviewer asked me to find the relationship between LCA and nodes. BTW N and Q values are upto 100000.
– zuccy
Nov 26 '18 at 5:08
@zuccy: Did the interviewer say anything about how the graph is represented?
– ruakh
Nov 26 '18 at 5:56
@zuccy: Did the interviewer say anything about how the graph is represented?
– ruakh
Nov 26 '18 at 5:56
Thank you senpai.
– zuccy
Nov 26 '18 at 18:10
Thank you senpai.
– zuccy
Nov 26 '18 at 18:10
add a comment |
Thanks for contributing an answer to Stack Overflow!
- Please be sure to answer the question. Provide details and share your research!
But avoid …
- Asking for help, clarification, or responding to other answers.
- Making statements based on opinion; back them up with references or personal experience.
To learn more, see our tips on writing great answers.
Sign up or log in
StackExchange.ready(function () {
StackExchange.helpers.onClickDraftSave('#login-link');
});
Sign up using Google
Sign up using Facebook
Sign up using Email and Password
Post as a guest
Required, but never shown
StackExchange.ready(
function () {
StackExchange.openid.initPostLogin('.new-post-login', 'https%3a%2f%2fstackoverflow.com%2fquestions%2f53450334%2ffind-most-visited-node-in-a-graph%23new-answer', 'question_page');
}
);
Post as a guest
Required, but never shown
Sign up or log in
StackExchange.ready(function () {
StackExchange.helpers.onClickDraftSave('#login-link');
});
Sign up using Google
Sign up using Facebook
Sign up using Email and Password
Post as a guest
Required, but never shown
Sign up or log in
StackExchange.ready(function () {
StackExchange.helpers.onClickDraftSave('#login-link');
});
Sign up using Google
Sign up using Facebook
Sign up using Email and Password
Post as a guest
Required, but never shown
Sign up or log in
StackExchange.ready(function () {
StackExchange.helpers.onClickDraftSave('#login-link');
});
Sign up using Google
Sign up using Facebook
Sign up using Email and Password
Sign up using Google
Sign up using Facebook
Sign up using Email and Password
Post as a guest
Required, but never shown
Required, but never shown
Required, but never shown
Required, but never shown
Required, but never shown
Required, but never shown
Required, but never shown
Required, but never shown
Required, but never shown
do H gcH7JzZ0xM0tTxEE,muBEP9zDC,bTQPRGrHD23E5wEb RCD9s6lJIAH2bF0oCg0j1N8kSh,dtNZ5Px6OcMPxf
Are you already given these Q paths or it is intention to inquire as less paths as possible to deduce which node is most visited? Result is one node or all nodes with same number of visits? I suppose that end nodes are also counted as visited?
– Ante
Nov 24 '18 at 7:32
Only source and destination nodes are given, not the paths. After travelling all those source to destination paths, some nodes will have max visited count. We need to find that count.
– zuccy
Nov 24 '18 at 7:57