Minimization problem solving and its step limits
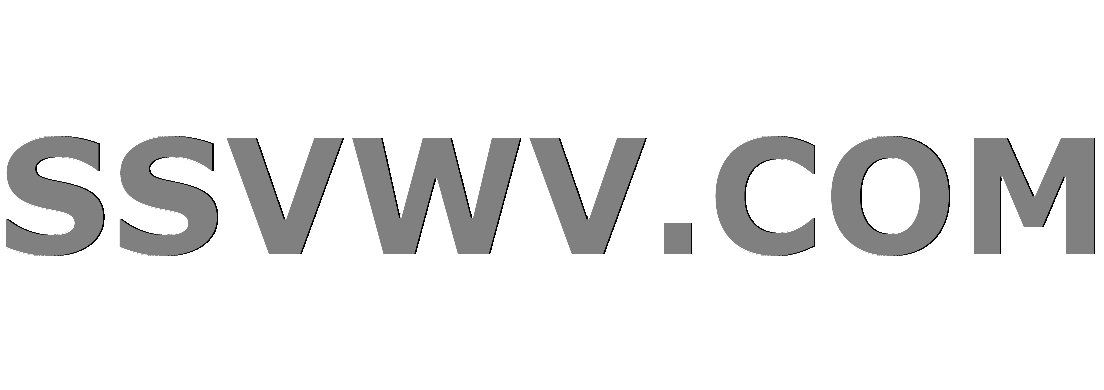
Multi tool use
I am trying to solve the following minimization problem:
$$
minlvertlvert{x}rvertrvert_1 + betalvertlvert{x}rvertrvert^2_2 s.t. sum_{m = 1}^m (y - lvert{c}^{H} . xrvert^2)^2) lg in
$$
x
: my unknown value (input) with complex elements and known size, here (4x1)
y
: the output vector (known)
c
: a 'skaling' vector
I am very new to this so my approach may seem basic. I simply loop over all combination of c
(non-redundant) and according to the computed minimization value and condition I update my results.
My questions are the following:
- Is this correct and is there a better approach to this?
- This code fails with a small step due to the huge size of combinations, so how can I solve that?
from itertools import combinations
from random import randint
import numpy as np
def deg2rad(phase):
return round(((phase*3.14)/180),3)
def excitation(amplitude, phase):
return complex(round(amplitude * (np.cos(deg2rad(phase))),3), round(amplitude*(np.sin(deg2rad(phase))),3))
def compute_subject_equation_result(x):
M = 12
difference =
y = [randint(10, 20) for i in range(M)]
for m in range(0, M):
c = np.array([randint(0, 9), randint(10,20), randint(0, 9), randint(0,20)]).reshape(4,1)
ch = c.conjugate().T
eq = (y[m] - (abs(np.dot(ch, x))[0])**2)**2
difference.append(eq**2)
return round(sum(difference)[0], 3)
def compute_main_equation_result(x, beta):
norm1 = np.linalg.norm(x,1)
norm2 = np.linalg.norm(x,2)
return round(norm1 + beta*(norm2**2), 3)
def optimize(x, min_x, min_phi_x):
min_result = 10**25
# compute the optimization formals and check for the min value
main_equation_result = compute_main_equation_result(c, beta)
subject_equation_result = compute_subject_equation_result(c)
# update min value if min detected'
if subject_equation_result < epsilon and main_equation_result < min_result:
min_result = main_equation_result
min_x = x
min_phi_x = phx
return min_x, min_phi_x
# initialization
phases = [alpha for alpha in range(0, 361, 90)]
beta = 1
epsilon = 10**25
min_x = np.array()
min_phi_x = np.array()
phases_combinations = [list(comb) for comb in combinations(phases, 4)]
# start checking all combinations
for phx in phases_combinations:
phi1, phi2, phi3, phi4 = phx[0], phx[1], phx[2], phx[3]
# build the hypothesis for the excitations vector c
c = np.array([ excitation(1, phi1), excitation(1, phi2), excitation(1, phi3), excitation(1, phi4) ]).T.reshape(4,1)
min_x, min_phi_x = optimize(c, min_x, min_phi_x)
print(' --------------------------------------------------')
print('-----> new_min_c = ', list(min_x))
print('-----> new_min_phi_c = ', min_phi_x)
Remark: When trying phases = [alpha for alpha in range(0, 361, 1)]
I get a "memory error". I can avoid using a higher step. However I am not sure about my approach in general nor of the step change effect on the accuracy.
python memory-optimization
New contributor
kogito is a new contributor to this site. Take care in asking for clarification, commenting, and answering.
Check out our Code of Conduct.
add a comment |
I am trying to solve the following minimization problem:
$$
minlvertlvert{x}rvertrvert_1 + betalvertlvert{x}rvertrvert^2_2 s.t. sum_{m = 1}^m (y - lvert{c}^{H} . xrvert^2)^2) lg in
$$
x
: my unknown value (input) with complex elements and known size, here (4x1)
y
: the output vector (known)
c
: a 'skaling' vector
I am very new to this so my approach may seem basic. I simply loop over all combination of c
(non-redundant) and according to the computed minimization value and condition I update my results.
My questions are the following:
- Is this correct and is there a better approach to this?
- This code fails with a small step due to the huge size of combinations, so how can I solve that?
from itertools import combinations
from random import randint
import numpy as np
def deg2rad(phase):
return round(((phase*3.14)/180),3)
def excitation(amplitude, phase):
return complex(round(amplitude * (np.cos(deg2rad(phase))),3), round(amplitude*(np.sin(deg2rad(phase))),3))
def compute_subject_equation_result(x):
M = 12
difference =
y = [randint(10, 20) for i in range(M)]
for m in range(0, M):
c = np.array([randint(0, 9), randint(10,20), randint(0, 9), randint(0,20)]).reshape(4,1)
ch = c.conjugate().T
eq = (y[m] - (abs(np.dot(ch, x))[0])**2)**2
difference.append(eq**2)
return round(sum(difference)[0], 3)
def compute_main_equation_result(x, beta):
norm1 = np.linalg.norm(x,1)
norm2 = np.linalg.norm(x,2)
return round(norm1 + beta*(norm2**2), 3)
def optimize(x, min_x, min_phi_x):
min_result = 10**25
# compute the optimization formals and check for the min value
main_equation_result = compute_main_equation_result(c, beta)
subject_equation_result = compute_subject_equation_result(c)
# update min value if min detected'
if subject_equation_result < epsilon and main_equation_result < min_result:
min_result = main_equation_result
min_x = x
min_phi_x = phx
return min_x, min_phi_x
# initialization
phases = [alpha for alpha in range(0, 361, 90)]
beta = 1
epsilon = 10**25
min_x = np.array()
min_phi_x = np.array()
phases_combinations = [list(comb) for comb in combinations(phases, 4)]
# start checking all combinations
for phx in phases_combinations:
phi1, phi2, phi3, phi4 = phx[0], phx[1], phx[2], phx[3]
# build the hypothesis for the excitations vector c
c = np.array([ excitation(1, phi1), excitation(1, phi2), excitation(1, phi3), excitation(1, phi4) ]).T.reshape(4,1)
min_x, min_phi_x = optimize(c, min_x, min_phi_x)
print(' --------------------------------------------------')
print('-----> new_min_c = ', list(min_x))
print('-----> new_min_phi_c = ', min_phi_x)
Remark: When trying phases = [alpha for alpha in range(0, 361, 1)]
I get a "memory error". I can avoid using a higher step. However I am not sure about my approach in general nor of the step change effect on the accuracy.
python memory-optimization
New contributor
kogito is a new contributor to this site. Take care in asking for clarification, commenting, and answering.
Check out our Code of Conduct.
add a comment |
I am trying to solve the following minimization problem:
$$
minlvertlvert{x}rvertrvert_1 + betalvertlvert{x}rvertrvert^2_2 s.t. sum_{m = 1}^m (y - lvert{c}^{H} . xrvert^2)^2) lg in
$$
x
: my unknown value (input) with complex elements and known size, here (4x1)
y
: the output vector (known)
c
: a 'skaling' vector
I am very new to this so my approach may seem basic. I simply loop over all combination of c
(non-redundant) and according to the computed minimization value and condition I update my results.
My questions are the following:
- Is this correct and is there a better approach to this?
- This code fails with a small step due to the huge size of combinations, so how can I solve that?
from itertools import combinations
from random import randint
import numpy as np
def deg2rad(phase):
return round(((phase*3.14)/180),3)
def excitation(amplitude, phase):
return complex(round(amplitude * (np.cos(deg2rad(phase))),3), round(amplitude*(np.sin(deg2rad(phase))),3))
def compute_subject_equation_result(x):
M = 12
difference =
y = [randint(10, 20) for i in range(M)]
for m in range(0, M):
c = np.array([randint(0, 9), randint(10,20), randint(0, 9), randint(0,20)]).reshape(4,1)
ch = c.conjugate().T
eq = (y[m] - (abs(np.dot(ch, x))[0])**2)**2
difference.append(eq**2)
return round(sum(difference)[0], 3)
def compute_main_equation_result(x, beta):
norm1 = np.linalg.norm(x,1)
norm2 = np.linalg.norm(x,2)
return round(norm1 + beta*(norm2**2), 3)
def optimize(x, min_x, min_phi_x):
min_result = 10**25
# compute the optimization formals and check for the min value
main_equation_result = compute_main_equation_result(c, beta)
subject_equation_result = compute_subject_equation_result(c)
# update min value if min detected'
if subject_equation_result < epsilon and main_equation_result < min_result:
min_result = main_equation_result
min_x = x
min_phi_x = phx
return min_x, min_phi_x
# initialization
phases = [alpha for alpha in range(0, 361, 90)]
beta = 1
epsilon = 10**25
min_x = np.array()
min_phi_x = np.array()
phases_combinations = [list(comb) for comb in combinations(phases, 4)]
# start checking all combinations
for phx in phases_combinations:
phi1, phi2, phi3, phi4 = phx[0], phx[1], phx[2], phx[3]
# build the hypothesis for the excitations vector c
c = np.array([ excitation(1, phi1), excitation(1, phi2), excitation(1, phi3), excitation(1, phi4) ]).T.reshape(4,1)
min_x, min_phi_x = optimize(c, min_x, min_phi_x)
print(' --------------------------------------------------')
print('-----> new_min_c = ', list(min_x))
print('-----> new_min_phi_c = ', min_phi_x)
Remark: When trying phases = [alpha for alpha in range(0, 361, 1)]
I get a "memory error". I can avoid using a higher step. However I am not sure about my approach in general nor of the step change effect on the accuracy.
python memory-optimization
New contributor
kogito is a new contributor to this site. Take care in asking for clarification, commenting, and answering.
Check out our Code of Conduct.
I am trying to solve the following minimization problem:
$$
minlvertlvert{x}rvertrvert_1 + betalvertlvert{x}rvertrvert^2_2 s.t. sum_{m = 1}^m (y - lvert{c}^{H} . xrvert^2)^2) lg in
$$
x
: my unknown value (input) with complex elements and known size, here (4x1)
y
: the output vector (known)
c
: a 'skaling' vector
I am very new to this so my approach may seem basic. I simply loop over all combination of c
(non-redundant) and according to the computed minimization value and condition I update my results.
My questions are the following:
- Is this correct and is there a better approach to this?
- This code fails with a small step due to the huge size of combinations, so how can I solve that?
from itertools import combinations
from random import randint
import numpy as np
def deg2rad(phase):
return round(((phase*3.14)/180),3)
def excitation(amplitude, phase):
return complex(round(amplitude * (np.cos(deg2rad(phase))),3), round(amplitude*(np.sin(deg2rad(phase))),3))
def compute_subject_equation_result(x):
M = 12
difference =
y = [randint(10, 20) for i in range(M)]
for m in range(0, M):
c = np.array([randint(0, 9), randint(10,20), randint(0, 9), randint(0,20)]).reshape(4,1)
ch = c.conjugate().T
eq = (y[m] - (abs(np.dot(ch, x))[0])**2)**2
difference.append(eq**2)
return round(sum(difference)[0], 3)
def compute_main_equation_result(x, beta):
norm1 = np.linalg.norm(x,1)
norm2 = np.linalg.norm(x,2)
return round(norm1 + beta*(norm2**2), 3)
def optimize(x, min_x, min_phi_x):
min_result = 10**25
# compute the optimization formals and check for the min value
main_equation_result = compute_main_equation_result(c, beta)
subject_equation_result = compute_subject_equation_result(c)
# update min value if min detected'
if subject_equation_result < epsilon and main_equation_result < min_result:
min_result = main_equation_result
min_x = x
min_phi_x = phx
return min_x, min_phi_x
# initialization
phases = [alpha for alpha in range(0, 361, 90)]
beta = 1
epsilon = 10**25
min_x = np.array()
min_phi_x = np.array()
phases_combinations = [list(comb) for comb in combinations(phases, 4)]
# start checking all combinations
for phx in phases_combinations:
phi1, phi2, phi3, phi4 = phx[0], phx[1], phx[2], phx[3]
# build the hypothesis for the excitations vector c
c = np.array([ excitation(1, phi1), excitation(1, phi2), excitation(1, phi3), excitation(1, phi4) ]).T.reshape(4,1)
min_x, min_phi_x = optimize(c, min_x, min_phi_x)
print(' --------------------------------------------------')
print('-----> new_min_c = ', list(min_x))
print('-----> new_min_phi_c = ', min_phi_x)
Remark: When trying phases = [alpha for alpha in range(0, 361, 1)]
I get a "memory error". I can avoid using a higher step. However I am not sure about my approach in general nor of the step change effect on the accuracy.
python memory-optimization
python memory-optimization
New contributor
kogito is a new contributor to this site. Take care in asking for clarification, commenting, and answering.
Check out our Code of Conduct.
New contributor
kogito is a new contributor to this site. Take care in asking for clarification, commenting, and answering.
Check out our Code of Conduct.
edited 23 mins ago


Jamal♦
30.3k11116226
30.3k11116226
New contributor
kogito is a new contributor to this site. Take care in asking for clarification, commenting, and answering.
Check out our Code of Conduct.
asked 1 hour ago
kogitokogito
1011
1011
New contributor
kogito is a new contributor to this site. Take care in asking for clarification, commenting, and answering.
Check out our Code of Conduct.
New contributor
kogito is a new contributor to this site. Take care in asking for clarification, commenting, and answering.
Check out our Code of Conduct.
kogito is a new contributor to this site. Take care in asking for clarification, commenting, and answering.
Check out our Code of Conduct.
add a comment |
add a comment |
0
active
oldest
votes
Your Answer
StackExchange.ifUsing("editor", function () {
return StackExchange.using("mathjaxEditing", function () {
StackExchange.MarkdownEditor.creationCallbacks.add(function (editor, postfix) {
StackExchange.mathjaxEditing.prepareWmdForMathJax(editor, postfix, [["\$", "\$"]]);
});
});
}, "mathjax-editing");
StackExchange.ifUsing("editor", function () {
StackExchange.using("externalEditor", function () {
StackExchange.using("snippets", function () {
StackExchange.snippets.init();
});
});
}, "code-snippets");
StackExchange.ready(function() {
var channelOptions = {
tags: "".split(" "),
id: "196"
};
initTagRenderer("".split(" "), "".split(" "), channelOptions);
StackExchange.using("externalEditor", function() {
// Have to fire editor after snippets, if snippets enabled
if (StackExchange.settings.snippets.snippetsEnabled) {
StackExchange.using("snippets", function() {
createEditor();
});
}
else {
createEditor();
}
});
function createEditor() {
StackExchange.prepareEditor({
heartbeatType: 'answer',
autoActivateHeartbeat: false,
convertImagesToLinks: false,
noModals: true,
showLowRepImageUploadWarning: true,
reputationToPostImages: null,
bindNavPrevention: true,
postfix: "",
imageUploader: {
brandingHtml: "Powered by u003ca class="icon-imgur-white" href="https://imgur.com/"u003eu003c/au003e",
contentPolicyHtml: "User contributions licensed under u003ca href="https://creativecommons.org/licenses/by-sa/3.0/"u003ecc by-sa 3.0 with attribution requiredu003c/au003e u003ca href="https://stackoverflow.com/legal/content-policy"u003e(content policy)u003c/au003e",
allowUrls: true
},
onDemand: true,
discardSelector: ".discard-answer"
,immediatelyShowMarkdownHelp:true
});
}
});
kogito is a new contributor. Be nice, and check out our Code of Conduct.
Sign up or log in
StackExchange.ready(function () {
StackExchange.helpers.onClickDraftSave('#login-link');
});
Sign up using Google
Sign up using Facebook
Sign up using Email and Password
Post as a guest
Required, but never shown
StackExchange.ready(
function () {
StackExchange.openid.initPostLogin('.new-post-login', 'https%3a%2f%2fcodereview.stackexchange.com%2fquestions%2f211153%2fminimization-problem-solving-and-its-step-limits%23new-answer', 'question_page');
}
);
Post as a guest
Required, but never shown
0
active
oldest
votes
0
active
oldest
votes
active
oldest
votes
active
oldest
votes
kogito is a new contributor. Be nice, and check out our Code of Conduct.
kogito is a new contributor. Be nice, and check out our Code of Conduct.
kogito is a new contributor. Be nice, and check out our Code of Conduct.
kogito is a new contributor. Be nice, and check out our Code of Conduct.
Thanks for contributing an answer to Code Review Stack Exchange!
- Please be sure to answer the question. Provide details and share your research!
But avoid …
- Asking for help, clarification, or responding to other answers.
- Making statements based on opinion; back them up with references or personal experience.
Use MathJax to format equations. MathJax reference.
To learn more, see our tips on writing great answers.
Some of your past answers have not been well-received, and you're in danger of being blocked from answering.
Please pay close attention to the following guidance:
- Please be sure to answer the question. Provide details and share your research!
But avoid …
- Asking for help, clarification, or responding to other answers.
- Making statements based on opinion; back them up with references or personal experience.
To learn more, see our tips on writing great answers.
Sign up or log in
StackExchange.ready(function () {
StackExchange.helpers.onClickDraftSave('#login-link');
});
Sign up using Google
Sign up using Facebook
Sign up using Email and Password
Post as a guest
Required, but never shown
StackExchange.ready(
function () {
StackExchange.openid.initPostLogin('.new-post-login', 'https%3a%2f%2fcodereview.stackexchange.com%2fquestions%2f211153%2fminimization-problem-solving-and-its-step-limits%23new-answer', 'question_page');
}
);
Post as a guest
Required, but never shown
Sign up or log in
StackExchange.ready(function () {
StackExchange.helpers.onClickDraftSave('#login-link');
});
Sign up using Google
Sign up using Facebook
Sign up using Email and Password
Post as a guest
Required, but never shown
Sign up or log in
StackExchange.ready(function () {
StackExchange.helpers.onClickDraftSave('#login-link');
});
Sign up using Google
Sign up using Facebook
Sign up using Email and Password
Post as a guest
Required, but never shown
Sign up or log in
StackExchange.ready(function () {
StackExchange.helpers.onClickDraftSave('#login-link');
});
Sign up using Google
Sign up using Facebook
Sign up using Email and Password
Sign up using Google
Sign up using Facebook
Sign up using Email and Password
Post as a guest
Required, but never shown
Required, but never shown
Required, but never shown
Required, but never shown
Required, but never shown
Required, but never shown
Required, but never shown
Required, but never shown
Required, but never shown
e,1O39w,hv0 6 rOU551o4wEZRusPyWhE Jc5Tc2Xh73uOPDWwUicHXcCot02Rp7 265wcsB8C,ddwQ6O2FGTBbaOb6