Save and continue training the LSTM network
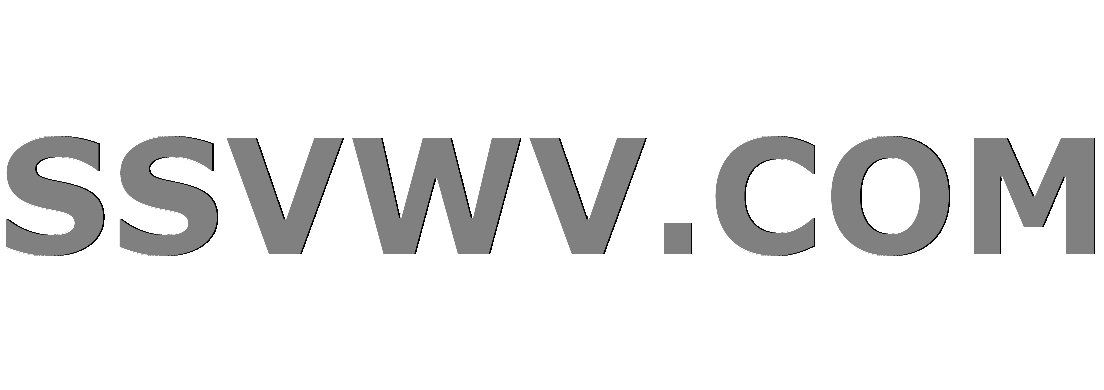
Multi tool use
I try to make a LSTM model continue were its last run left off. All compiles fine till I try to fit the network. Then it gives an error:
ValueError: Error when checking target: expected dense_29 to have 3 dimensions, but got array with shape (672, 1)
I checked various articles such as this and this but I don't see what is wrong in my code.
from keras import Sequential
from keras.preprocessing.sequence import pad_sequences
from sklearn.model_selection import train_test_split
from keras.models import Sequential,Model
from keras.layers import LSTM, Dense, Bidirectional, Input,Dropout,BatchNormalization
from keras import backend as K
from keras.engine.topology import Layer
from keras import initializers, regularizers, constraints
from keras.callbacks import ModelCheckpoint
from keras.models import load_model
import os.path
import os
filepath="Train-weights.best.hdf5"
act = 'relu'
model = Sequential()
model.add(BatchNormalization(input_shape=(10, 128)))
model.add(Bidirectional(LSTM(128, dropout=0.5, activation=act, return_sequences=True)))
model.add(Dense(1,activation='sigmoid'))
if (os.path.exists(filepath)):
print("extending training of previous run")
model.compile(loss='binary_crossentropy', optimizer='adam', metrics=['accuracy'])
with open('model_architecture.json', 'r') as f:
model = model_from_json(f.read())
model.load_weights(filepath)
else:
print("First run")
model.compile(loss='binary_crossentropy', optimizer='adam', metrics=['accuracy'])
model.fit(x_train, y_train, validation_data=(x_val, y_val), epochs=100, batch_size=32, callbacks=callbacks_list, verbose=2)
model.save_weights(filepath)
with open('model_architecture.json', 'w') as f:
f.write(model.to_json())
checkpoint = ModelCheckpoint(filepath, monitor='val_acc', verbose=1, save_best_only=True, mode='max')
callbacks_list = [checkpoint]
model.fit(x_train, y_train, validation_data=(x_val, y_val), epochs=100, batch_size=32, callbacks=callbacks_list, verbose=0)
python machine-learning keras lstm recurrent-neural-network
add a comment |
I try to make a LSTM model continue were its last run left off. All compiles fine till I try to fit the network. Then it gives an error:
ValueError: Error when checking target: expected dense_29 to have 3 dimensions, but got array with shape (672, 1)
I checked various articles such as this and this but I don't see what is wrong in my code.
from keras import Sequential
from keras.preprocessing.sequence import pad_sequences
from sklearn.model_selection import train_test_split
from keras.models import Sequential,Model
from keras.layers import LSTM, Dense, Bidirectional, Input,Dropout,BatchNormalization
from keras import backend as K
from keras.engine.topology import Layer
from keras import initializers, regularizers, constraints
from keras.callbacks import ModelCheckpoint
from keras.models import load_model
import os.path
import os
filepath="Train-weights.best.hdf5"
act = 'relu'
model = Sequential()
model.add(BatchNormalization(input_shape=(10, 128)))
model.add(Bidirectional(LSTM(128, dropout=0.5, activation=act, return_sequences=True)))
model.add(Dense(1,activation='sigmoid'))
if (os.path.exists(filepath)):
print("extending training of previous run")
model.compile(loss='binary_crossentropy', optimizer='adam', metrics=['accuracy'])
with open('model_architecture.json', 'r') as f:
model = model_from_json(f.read())
model.load_weights(filepath)
else:
print("First run")
model.compile(loss='binary_crossentropy', optimizer='adam', metrics=['accuracy'])
model.fit(x_train, y_train, validation_data=(x_val, y_val), epochs=100, batch_size=32, callbacks=callbacks_list, verbose=2)
model.save_weights(filepath)
with open('model_architecture.json', 'w') as f:
f.write(model.to_json())
checkpoint = ModelCheckpoint(filepath, monitor='val_acc', verbose=1, save_best_only=True, mode='max')
callbacks_list = [checkpoint]
model.fit(x_train, y_train, validation_data=(x_val, y_val), epochs=100, batch_size=32, callbacks=callbacks_list, verbose=0)
python machine-learning keras lstm recurrent-neural-network
add a comment |
I try to make a LSTM model continue were its last run left off. All compiles fine till I try to fit the network. Then it gives an error:
ValueError: Error when checking target: expected dense_29 to have 3 dimensions, but got array with shape (672, 1)
I checked various articles such as this and this but I don't see what is wrong in my code.
from keras import Sequential
from keras.preprocessing.sequence import pad_sequences
from sklearn.model_selection import train_test_split
from keras.models import Sequential,Model
from keras.layers import LSTM, Dense, Bidirectional, Input,Dropout,BatchNormalization
from keras import backend as K
from keras.engine.topology import Layer
from keras import initializers, regularizers, constraints
from keras.callbacks import ModelCheckpoint
from keras.models import load_model
import os.path
import os
filepath="Train-weights.best.hdf5"
act = 'relu'
model = Sequential()
model.add(BatchNormalization(input_shape=(10, 128)))
model.add(Bidirectional(LSTM(128, dropout=0.5, activation=act, return_sequences=True)))
model.add(Dense(1,activation='sigmoid'))
if (os.path.exists(filepath)):
print("extending training of previous run")
model.compile(loss='binary_crossentropy', optimizer='adam', metrics=['accuracy'])
with open('model_architecture.json', 'r') as f:
model = model_from_json(f.read())
model.load_weights(filepath)
else:
print("First run")
model.compile(loss='binary_crossentropy', optimizer='adam', metrics=['accuracy'])
model.fit(x_train, y_train, validation_data=(x_val, y_val), epochs=100, batch_size=32, callbacks=callbacks_list, verbose=2)
model.save_weights(filepath)
with open('model_architecture.json', 'w') as f:
f.write(model.to_json())
checkpoint = ModelCheckpoint(filepath, monitor='val_acc', verbose=1, save_best_only=True, mode='max')
callbacks_list = [checkpoint]
model.fit(x_train, y_train, validation_data=(x_val, y_val), epochs=100, batch_size=32, callbacks=callbacks_list, verbose=0)
python machine-learning keras lstm recurrent-neural-network
I try to make a LSTM model continue were its last run left off. All compiles fine till I try to fit the network. Then it gives an error:
ValueError: Error when checking target: expected dense_29 to have 3 dimensions, but got array with shape (672, 1)
I checked various articles such as this and this but I don't see what is wrong in my code.
from keras import Sequential
from keras.preprocessing.sequence import pad_sequences
from sklearn.model_selection import train_test_split
from keras.models import Sequential,Model
from keras.layers import LSTM, Dense, Bidirectional, Input,Dropout,BatchNormalization
from keras import backend as K
from keras.engine.topology import Layer
from keras import initializers, regularizers, constraints
from keras.callbacks import ModelCheckpoint
from keras.models import load_model
import os.path
import os
filepath="Train-weights.best.hdf5"
act = 'relu'
model = Sequential()
model.add(BatchNormalization(input_shape=(10, 128)))
model.add(Bidirectional(LSTM(128, dropout=0.5, activation=act, return_sequences=True)))
model.add(Dense(1,activation='sigmoid'))
if (os.path.exists(filepath)):
print("extending training of previous run")
model.compile(loss='binary_crossentropy', optimizer='adam', metrics=['accuracy'])
with open('model_architecture.json', 'r') as f:
model = model_from_json(f.read())
model.load_weights(filepath)
else:
print("First run")
model.compile(loss='binary_crossentropy', optimizer='adam', metrics=['accuracy'])
model.fit(x_train, y_train, validation_data=(x_val, y_val), epochs=100, batch_size=32, callbacks=callbacks_list, verbose=2)
model.save_weights(filepath)
with open('model_architecture.json', 'w') as f:
f.write(model.to_json())
checkpoint = ModelCheckpoint(filepath, monitor='val_acc', verbose=1, save_best_only=True, mode='max')
callbacks_list = [checkpoint]
model.fit(x_train, y_train, validation_data=(x_val, y_val), epochs=100, batch_size=32, callbacks=callbacks_list, verbose=0)
python machine-learning keras lstm recurrent-neural-network
python machine-learning keras lstm recurrent-neural-network
edited Nov 21 '18 at 13:59
today
9,97621536
9,97621536
asked Nov 21 '18 at 13:47
user3800527
757721
757721
add a comment |
add a comment |
1 Answer
1
active
oldest
votes
Try model.summary()
and you would see that the output shape of last layer (i.e. the Dense layer) in the network is (None, 10, 1)
. Therefore, the labels you provide to the model (i.e. y_train
) must also have a shape of (num_samples, 10, 1)
.
If the output shape (None, 10, 1)
is not what you wanted (e.g. you want (None, 1)
as the output shape of your model) then you need to modify your model definition. One simple modification to achieve that is removing the return_sequences=True
argument from the LSTM layer.
I missed it return_sequences... thanks.
– user3800527
Nov 21 '18 at 18:47
add a comment |
Your Answer
StackExchange.ifUsing("editor", function () {
StackExchange.using("externalEditor", function () {
StackExchange.using("snippets", function () {
StackExchange.snippets.init();
});
});
}, "code-snippets");
StackExchange.ready(function() {
var channelOptions = {
tags: "".split(" "),
id: "1"
};
initTagRenderer("".split(" "), "".split(" "), channelOptions);
StackExchange.using("externalEditor", function() {
// Have to fire editor after snippets, if snippets enabled
if (StackExchange.settings.snippets.snippetsEnabled) {
StackExchange.using("snippets", function() {
createEditor();
});
}
else {
createEditor();
}
});
function createEditor() {
StackExchange.prepareEditor({
heartbeatType: 'answer',
autoActivateHeartbeat: false,
convertImagesToLinks: true,
noModals: true,
showLowRepImageUploadWarning: true,
reputationToPostImages: 10,
bindNavPrevention: true,
postfix: "",
imageUploader: {
brandingHtml: "Powered by u003ca class="icon-imgur-white" href="https://imgur.com/"u003eu003c/au003e",
contentPolicyHtml: "User contributions licensed under u003ca href="https://creativecommons.org/licenses/by-sa/3.0/"u003ecc by-sa 3.0 with attribution requiredu003c/au003e u003ca href="https://stackoverflow.com/legal/content-policy"u003e(content policy)u003c/au003e",
allowUrls: true
},
onDemand: true,
discardSelector: ".discard-answer"
,immediatelyShowMarkdownHelp:true
});
}
});
Sign up or log in
StackExchange.ready(function () {
StackExchange.helpers.onClickDraftSave('#login-link');
});
Sign up using Google
Sign up using Facebook
Sign up using Email and Password
Post as a guest
Required, but never shown
StackExchange.ready(
function () {
StackExchange.openid.initPostLogin('.new-post-login', 'https%3a%2f%2fstackoverflow.com%2fquestions%2f53413505%2fsave-and-continue-training-the-lstm-network%23new-answer', 'question_page');
}
);
Post as a guest
Required, but never shown
1 Answer
1
active
oldest
votes
1 Answer
1
active
oldest
votes
active
oldest
votes
active
oldest
votes
Try model.summary()
and you would see that the output shape of last layer (i.e. the Dense layer) in the network is (None, 10, 1)
. Therefore, the labels you provide to the model (i.e. y_train
) must also have a shape of (num_samples, 10, 1)
.
If the output shape (None, 10, 1)
is not what you wanted (e.g. you want (None, 1)
as the output shape of your model) then you need to modify your model definition. One simple modification to achieve that is removing the return_sequences=True
argument from the LSTM layer.
I missed it return_sequences... thanks.
– user3800527
Nov 21 '18 at 18:47
add a comment |
Try model.summary()
and you would see that the output shape of last layer (i.e. the Dense layer) in the network is (None, 10, 1)
. Therefore, the labels you provide to the model (i.e. y_train
) must also have a shape of (num_samples, 10, 1)
.
If the output shape (None, 10, 1)
is not what you wanted (e.g. you want (None, 1)
as the output shape of your model) then you need to modify your model definition. One simple modification to achieve that is removing the return_sequences=True
argument from the LSTM layer.
I missed it return_sequences... thanks.
– user3800527
Nov 21 '18 at 18:47
add a comment |
Try model.summary()
and you would see that the output shape of last layer (i.e. the Dense layer) in the network is (None, 10, 1)
. Therefore, the labels you provide to the model (i.e. y_train
) must also have a shape of (num_samples, 10, 1)
.
If the output shape (None, 10, 1)
is not what you wanted (e.g. you want (None, 1)
as the output shape of your model) then you need to modify your model definition. One simple modification to achieve that is removing the return_sequences=True
argument from the LSTM layer.
Try model.summary()
and you would see that the output shape of last layer (i.e. the Dense layer) in the network is (None, 10, 1)
. Therefore, the labels you provide to the model (i.e. y_train
) must also have a shape of (num_samples, 10, 1)
.
If the output shape (None, 10, 1)
is not what you wanted (e.g. you want (None, 1)
as the output shape of your model) then you need to modify your model definition. One simple modification to achieve that is removing the return_sequences=True
argument from the LSTM layer.
answered Nov 21 '18 at 13:56
today
9,97621536
9,97621536
I missed it return_sequences... thanks.
– user3800527
Nov 21 '18 at 18:47
add a comment |
I missed it return_sequences... thanks.
– user3800527
Nov 21 '18 at 18:47
I missed it return_sequences... thanks.
– user3800527
Nov 21 '18 at 18:47
I missed it return_sequences... thanks.
– user3800527
Nov 21 '18 at 18:47
add a comment |
Thanks for contributing an answer to Stack Overflow!
- Please be sure to answer the question. Provide details and share your research!
But avoid …
- Asking for help, clarification, or responding to other answers.
- Making statements based on opinion; back them up with references or personal experience.
To learn more, see our tips on writing great answers.
Some of your past answers have not been well-received, and you're in danger of being blocked from answering.
Please pay close attention to the following guidance:
- Please be sure to answer the question. Provide details and share your research!
But avoid …
- Asking for help, clarification, or responding to other answers.
- Making statements based on opinion; back them up with references or personal experience.
To learn more, see our tips on writing great answers.
Sign up or log in
StackExchange.ready(function () {
StackExchange.helpers.onClickDraftSave('#login-link');
});
Sign up using Google
Sign up using Facebook
Sign up using Email and Password
Post as a guest
Required, but never shown
StackExchange.ready(
function () {
StackExchange.openid.initPostLogin('.new-post-login', 'https%3a%2f%2fstackoverflow.com%2fquestions%2f53413505%2fsave-and-continue-training-the-lstm-network%23new-answer', 'question_page');
}
);
Post as a guest
Required, but never shown
Sign up or log in
StackExchange.ready(function () {
StackExchange.helpers.onClickDraftSave('#login-link');
});
Sign up using Google
Sign up using Facebook
Sign up using Email and Password
Post as a guest
Required, but never shown
Sign up or log in
StackExchange.ready(function () {
StackExchange.helpers.onClickDraftSave('#login-link');
});
Sign up using Google
Sign up using Facebook
Sign up using Email and Password
Post as a guest
Required, but never shown
Sign up or log in
StackExchange.ready(function () {
StackExchange.helpers.onClickDraftSave('#login-link');
});
Sign up using Google
Sign up using Facebook
Sign up using Email and Password
Sign up using Google
Sign up using Facebook
Sign up using Email and Password
Post as a guest
Required, but never shown
Required, but never shown
Required, but never shown
Required, but never shown
Required, but never shown
Required, but never shown
Required, but never shown
Required, but never shown
Required, but never shown
RbsQ yu,j,o7wUJvG,2NYB8 FUKrf 28r7,klMBJVdCG