find maximum value in col C in pandas dataframe while group by both col A and B
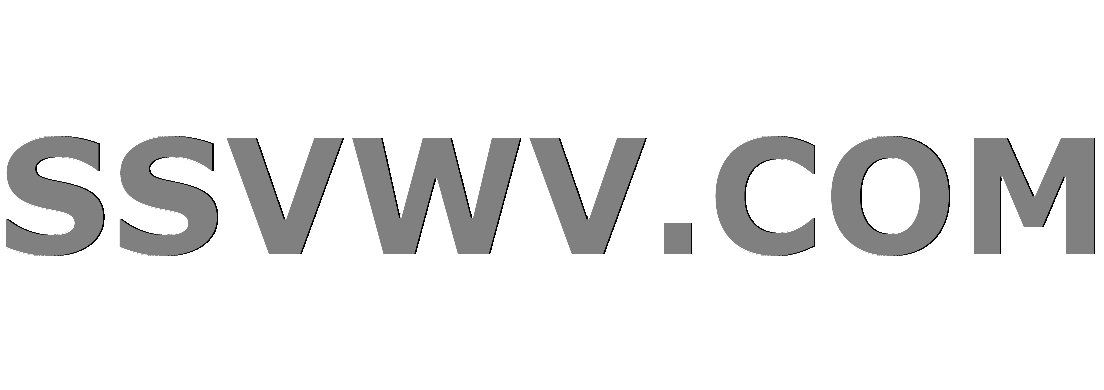
Multi tool use
up vote
0
down vote
favorite
I have a pandas dataframe like this:
df = pd.DataFrame({"RT":[9,10,10,11,11,11,11],"Quality":[70,60,50,60,80,70,80],'Name' :['a','a','b','c','b','c','b'],'Similarity':[0.98,0.97,0.97,0.95,0.95,0.95,0.95]})
RT Quality Name Similarity
0 9 70 a 0.98
1 10 60 a 0.97
2 10 50 b 0.97
3 11 60 c 0.95
4 11 80 b 0.95
5 11 70 c 0.95
6 11 80 b 0.95
The values in the column Similarity
has the same group-by with column RT
I want to group column RT
and find the maximum column Quality
value and group by column Name
.
For example:
In column RT
value 11
,which have column Name
value c
and b
, sum each of the column Quality
values, then get c = 130, b =160
, and sort the maximum 160, b
then get
RT Quality Name Similarity
0 9 70 a 0.98
1 10 60 a 0.97
2 10 50 b 0.97
3 11 160 b 0.95
4 11 130 c 0.95
python pandas
add a comment |
up vote
0
down vote
favorite
I have a pandas dataframe like this:
df = pd.DataFrame({"RT":[9,10,10,11,11,11,11],"Quality":[70,60,50,60,80,70,80],'Name' :['a','a','b','c','b','c','b'],'Similarity':[0.98,0.97,0.97,0.95,0.95,0.95,0.95]})
RT Quality Name Similarity
0 9 70 a 0.98
1 10 60 a 0.97
2 10 50 b 0.97
3 11 60 c 0.95
4 11 80 b 0.95
5 11 70 c 0.95
6 11 80 b 0.95
The values in the column Similarity
has the same group-by with column RT
I want to group column RT
and find the maximum column Quality
value and group by column Name
.
For example:
In column RT
value 11
,which have column Name
value c
and b
, sum each of the column Quality
values, then get c = 130, b =160
, and sort the maximum 160, b
then get
RT Quality Name Similarity
0 9 70 a 0.98
1 10 60 a 0.97
2 10 50 b 0.97
3 11 160 b 0.95
4 11 130 c 0.95
python pandas
add a comment |
up vote
0
down vote
favorite
up vote
0
down vote
favorite
I have a pandas dataframe like this:
df = pd.DataFrame({"RT":[9,10,10,11,11,11,11],"Quality":[70,60,50,60,80,70,80],'Name' :['a','a','b','c','b','c','b'],'Similarity':[0.98,0.97,0.97,0.95,0.95,0.95,0.95]})
RT Quality Name Similarity
0 9 70 a 0.98
1 10 60 a 0.97
2 10 50 b 0.97
3 11 60 c 0.95
4 11 80 b 0.95
5 11 70 c 0.95
6 11 80 b 0.95
The values in the column Similarity
has the same group-by with column RT
I want to group column RT
and find the maximum column Quality
value and group by column Name
.
For example:
In column RT
value 11
,which have column Name
value c
and b
, sum each of the column Quality
values, then get c = 130, b =160
, and sort the maximum 160, b
then get
RT Quality Name Similarity
0 9 70 a 0.98
1 10 60 a 0.97
2 10 50 b 0.97
3 11 160 b 0.95
4 11 130 c 0.95
python pandas
I have a pandas dataframe like this:
df = pd.DataFrame({"RT":[9,10,10,11,11,11,11],"Quality":[70,60,50,60,80,70,80],'Name' :['a','a','b','c','b','c','b'],'Similarity':[0.98,0.97,0.97,0.95,0.95,0.95,0.95]})
RT Quality Name Similarity
0 9 70 a 0.98
1 10 60 a 0.97
2 10 50 b 0.97
3 11 60 c 0.95
4 11 80 b 0.95
5 11 70 c 0.95
6 11 80 b 0.95
The values in the column Similarity
has the same group-by with column RT
I want to group column RT
and find the maximum column Quality
value and group by column Name
.
For example:
In column RT
value 11
,which have column Name
value c
and b
, sum each of the column Quality
values, then get c = 130, b =160
, and sort the maximum 160, b
then get
RT Quality Name Similarity
0 9 70 a 0.98
1 10 60 a 0.97
2 10 50 b 0.97
3 11 160 b 0.95
4 11 130 c 0.95
python pandas
python pandas
edited Nov 20 at 7:33
ssemilla
2,687423
2,687423
asked Nov 20 at 2:27
X.tang
93
93
add a comment |
add a comment |
2 Answers
2
active
oldest
votes
up vote
1
down vote
you can use groupby with agg:
use lambda to return all Similarities or max to return the max
df.groupby(['RT','Name']).agg({'Quality':'sum', 'Similarity':lambda x:x.unique()})
Quality Similarity
RT Name
9 a 70 0.98
10 a 60 0.97
b 50 0.97
11 b 160 0.95
c 130 0.95
thank you very much~
– X.tang
Nov 21 at 11:49
add a comment |
up vote
0
down vote
You may not need agg
df.groupby(['RT','Similarity','Name'],as_index=False)['Quality'].sum()
Out[150]:
RT Similarity Name Quality
0 9 0.98 a 70
1 10 0.97 a 60
2 10 0.97 b 50
3 11 0.95 b 160
4 11 0.95 c 130
Thank you for your reply, what is the difference betweenagg
andas_index
? and if I have some irrelevant column,how to keep those column use your method .df = pd.DataFrame({"RT":[9,10,10,11,11,11,11],"Quality":[70,60,50,60,80,70,80],'Name' :['a','a','b','c','b','c','b'], 'Similarity':[0.98,0.97,0.97,0.95,0.95,0.95,0.95],"samples":[13,2,4,5,6,6,7,]})
such as keep the columnsamples
along with column['RT','Similarity','Name']
– X.tang
Nov 21 at 11:47
add a comment |
2 Answers
2
active
oldest
votes
2 Answers
2
active
oldest
votes
active
oldest
votes
active
oldest
votes
up vote
1
down vote
you can use groupby with agg:
use lambda to return all Similarities or max to return the max
df.groupby(['RT','Name']).agg({'Quality':'sum', 'Similarity':lambda x:x.unique()})
Quality Similarity
RT Name
9 a 70 0.98
10 a 60 0.97
b 50 0.97
11 b 160 0.95
c 130 0.95
thank you very much~
– X.tang
Nov 21 at 11:49
add a comment |
up vote
1
down vote
you can use groupby with agg:
use lambda to return all Similarities or max to return the max
df.groupby(['RT','Name']).agg({'Quality':'sum', 'Similarity':lambda x:x.unique()})
Quality Similarity
RT Name
9 a 70 0.98
10 a 60 0.97
b 50 0.97
11 b 160 0.95
c 130 0.95
thank you very much~
– X.tang
Nov 21 at 11:49
add a comment |
up vote
1
down vote
up vote
1
down vote
you can use groupby with agg:
use lambda to return all Similarities or max to return the max
df.groupby(['RT','Name']).agg({'Quality':'sum', 'Similarity':lambda x:x.unique()})
Quality Similarity
RT Name
9 a 70 0.98
10 a 60 0.97
b 50 0.97
11 b 160 0.95
c 130 0.95
you can use groupby with agg:
use lambda to return all Similarities or max to return the max
df.groupby(['RT','Name']).agg({'Quality':'sum', 'Similarity':lambda x:x.unique()})
Quality Similarity
RT Name
9 a 70 0.98
10 a 60 0.97
b 50 0.97
11 b 160 0.95
c 130 0.95
edited Nov 20 at 2:56
answered Nov 20 at 2:36
Chris
1,3731210
1,3731210
thank you very much~
– X.tang
Nov 21 at 11:49
add a comment |
thank you very much~
– X.tang
Nov 21 at 11:49
thank you very much~
– X.tang
Nov 21 at 11:49
thank you very much~
– X.tang
Nov 21 at 11:49
add a comment |
up vote
0
down vote
You may not need agg
df.groupby(['RT','Similarity','Name'],as_index=False)['Quality'].sum()
Out[150]:
RT Similarity Name Quality
0 9 0.98 a 70
1 10 0.97 a 60
2 10 0.97 b 50
3 11 0.95 b 160
4 11 0.95 c 130
Thank you for your reply, what is the difference betweenagg
andas_index
? and if I have some irrelevant column,how to keep those column use your method .df = pd.DataFrame({"RT":[9,10,10,11,11,11,11],"Quality":[70,60,50,60,80,70,80],'Name' :['a','a','b','c','b','c','b'], 'Similarity':[0.98,0.97,0.97,0.95,0.95,0.95,0.95],"samples":[13,2,4,5,6,6,7,]})
such as keep the columnsamples
along with column['RT','Similarity','Name']
– X.tang
Nov 21 at 11:47
add a comment |
up vote
0
down vote
You may not need agg
df.groupby(['RT','Similarity','Name'],as_index=False)['Quality'].sum()
Out[150]:
RT Similarity Name Quality
0 9 0.98 a 70
1 10 0.97 a 60
2 10 0.97 b 50
3 11 0.95 b 160
4 11 0.95 c 130
Thank you for your reply, what is the difference betweenagg
andas_index
? and if I have some irrelevant column,how to keep those column use your method .df = pd.DataFrame({"RT":[9,10,10,11,11,11,11],"Quality":[70,60,50,60,80,70,80],'Name' :['a','a','b','c','b','c','b'], 'Similarity':[0.98,0.97,0.97,0.95,0.95,0.95,0.95],"samples":[13,2,4,5,6,6,7,]})
such as keep the columnsamples
along with column['RT','Similarity','Name']
– X.tang
Nov 21 at 11:47
add a comment |
up vote
0
down vote
up vote
0
down vote
You may not need agg
df.groupby(['RT','Similarity','Name'],as_index=False)['Quality'].sum()
Out[150]:
RT Similarity Name Quality
0 9 0.98 a 70
1 10 0.97 a 60
2 10 0.97 b 50
3 11 0.95 b 160
4 11 0.95 c 130
You may not need agg
df.groupby(['RT','Similarity','Name'],as_index=False)['Quality'].sum()
Out[150]:
RT Similarity Name Quality
0 9 0.98 a 70
1 10 0.97 a 60
2 10 0.97 b 50
3 11 0.95 b 160
4 11 0.95 c 130
answered Nov 20 at 4:26


W-B
96.3k72962
96.3k72962
Thank you for your reply, what is the difference betweenagg
andas_index
? and if I have some irrelevant column,how to keep those column use your method .df = pd.DataFrame({"RT":[9,10,10,11,11,11,11],"Quality":[70,60,50,60,80,70,80],'Name' :['a','a','b','c','b','c','b'], 'Similarity':[0.98,0.97,0.97,0.95,0.95,0.95,0.95],"samples":[13,2,4,5,6,6,7,]})
such as keep the columnsamples
along with column['RT','Similarity','Name']
– X.tang
Nov 21 at 11:47
add a comment |
Thank you for your reply, what is the difference betweenagg
andas_index
? and if I have some irrelevant column,how to keep those column use your method .df = pd.DataFrame({"RT":[9,10,10,11,11,11,11],"Quality":[70,60,50,60,80,70,80],'Name' :['a','a','b','c','b','c','b'], 'Similarity':[0.98,0.97,0.97,0.95,0.95,0.95,0.95],"samples":[13,2,4,5,6,6,7,]})
such as keep the columnsamples
along with column['RT','Similarity','Name']
– X.tang
Nov 21 at 11:47
Thank you for your reply, what is the difference between
agg
and as_index
? and if I have some irrelevant column,how to keep those column use your method . df = pd.DataFrame({"RT":[9,10,10,11,11,11,11],"Quality":[70,60,50,60,80,70,80],'Name' :['a','a','b','c','b','c','b'], 'Similarity':[0.98,0.97,0.97,0.95,0.95,0.95,0.95],"samples":[13,2,4,5,6,6,7,]})
such as keep the column samples
along with column ['RT','Similarity','Name']
– X.tang
Nov 21 at 11:47
Thank you for your reply, what is the difference between
agg
and as_index
? and if I have some irrelevant column,how to keep those column use your method . df = pd.DataFrame({"RT":[9,10,10,11,11,11,11],"Quality":[70,60,50,60,80,70,80],'Name' :['a','a','b','c','b','c','b'], 'Similarity':[0.98,0.97,0.97,0.95,0.95,0.95,0.95],"samples":[13,2,4,5,6,6,7,]})
such as keep the column samples
along with column ['RT','Similarity','Name']
– X.tang
Nov 21 at 11:47
add a comment |
Thanks for contributing an answer to Stack Overflow!
- Please be sure to answer the question. Provide details and share your research!
But avoid …
- Asking for help, clarification, or responding to other answers.
- Making statements based on opinion; back them up with references or personal experience.
To learn more, see our tips on writing great answers.
Some of your past answers have not been well-received, and you're in danger of being blocked from answering.
Please pay close attention to the following guidance:
- Please be sure to answer the question. Provide details and share your research!
But avoid …
- Asking for help, clarification, or responding to other answers.
- Making statements based on opinion; back them up with references or personal experience.
To learn more, see our tips on writing great answers.
Sign up or log in
StackExchange.ready(function () {
StackExchange.helpers.onClickDraftSave('#login-link');
});
Sign up using Google
Sign up using Facebook
Sign up using Email and Password
Post as a guest
Required, but never shown
StackExchange.ready(
function () {
StackExchange.openid.initPostLogin('.new-post-login', 'https%3a%2f%2fstackoverflow.com%2fquestions%2f53385348%2ffind-maximum-value-in-col-c-in-pandas-dataframe-while-group-by-both-col-a-and-b%23new-answer', 'question_page');
}
);
Post as a guest
Required, but never shown
Sign up or log in
StackExchange.ready(function () {
StackExchange.helpers.onClickDraftSave('#login-link');
});
Sign up using Google
Sign up using Facebook
Sign up using Email and Password
Post as a guest
Required, but never shown
Sign up or log in
StackExchange.ready(function () {
StackExchange.helpers.onClickDraftSave('#login-link');
});
Sign up using Google
Sign up using Facebook
Sign up using Email and Password
Post as a guest
Required, but never shown
Sign up or log in
StackExchange.ready(function () {
StackExchange.helpers.onClickDraftSave('#login-link');
});
Sign up using Google
Sign up using Facebook
Sign up using Email and Password
Sign up using Google
Sign up using Facebook
Sign up using Email and Password
Post as a guest
Required, but never shown
Required, but never shown
Required, but never shown
Required, but never shown
Required, but never shown
Required, but never shown
Required, but never shown
Required, but never shown
Required, but never shown
jtwLC82Qa J CivV2Lj0YvG,EdG1pN6,68VPugzS9Nt,A93 EYu2aP,rA8O9WVr