Dataflow/apache beam - how to access current filename when passing in pattern?
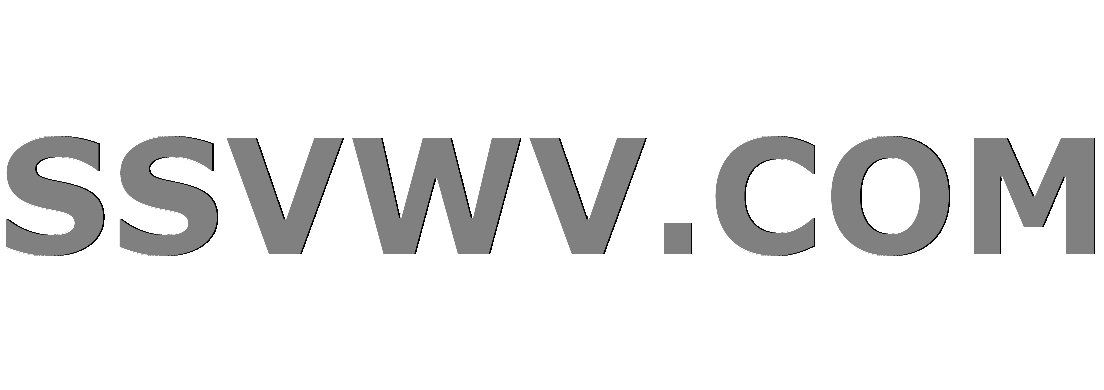
Multi tool use
I have seen this question answered before on stack overflow (https://stackoverflow.com/questions/29983621/how-to-get-filename-when-using-file-pattern-match-in-google-cloud-dataflow), but not since apache beam has added splittable dofn functionality for python. How would I access the filename of the current file being processed when passing in a file pattern to a gcs bucket?
I want to pass the filename into my transform function:
with beam.Pipeline(options=pipeline_options) as p:
lines = p | ReadFromText('gs://url to file')
data = (
lines
| 'Jsonify' >> beam.Map(jsonify)
| 'Unnest' >> beam.FlatMap(unnest)
| 'Write to BQ' >> beam.io.Write(beam.io.BigQuerySink(
'project_id:dataset_id.table_name', schema=schema,
create_disposition=beam.io.BigQueryDisposition.CREATE_IF_NEEDED,
write_disposition=beam.io.BigQueryDisposition.WRITE_APPEND)
)
Ultimately, what I want to do is pass the filename into my transform function when I transform each row of the json (see this and then use the filename to do a lookup in a different BQ table to get a value). I think once I manage to know how to get the filename I will be able to figure out the side input part in order to do the lookup in the bq table and get the unique value.
python



add a comment |
I have seen this question answered before on stack overflow (https://stackoverflow.com/questions/29983621/how-to-get-filename-when-using-file-pattern-match-in-google-cloud-dataflow), but not since apache beam has added splittable dofn functionality for python. How would I access the filename of the current file being processed when passing in a file pattern to a gcs bucket?
I want to pass the filename into my transform function:
with beam.Pipeline(options=pipeline_options) as p:
lines = p | ReadFromText('gs://url to file')
data = (
lines
| 'Jsonify' >> beam.Map(jsonify)
| 'Unnest' >> beam.FlatMap(unnest)
| 'Write to BQ' >> beam.io.Write(beam.io.BigQuerySink(
'project_id:dataset_id.table_name', schema=schema,
create_disposition=beam.io.BigQueryDisposition.CREATE_IF_NEEDED,
write_disposition=beam.io.BigQueryDisposition.WRITE_APPEND)
)
Ultimately, what I want to do is pass the filename into my transform function when I transform each row of the json (see this and then use the filename to do a lookup in a different BQ table to get a value). I think once I manage to know how to get the filename I will be able to figure out the side input part in order to do the lookup in the bq table and get the unique value.
python



1
You can take a look at this (mind the solution in the question too)
– Guillem Xercavins
Nov 23 at 19:29
@GuillemXercavins your example is reading an entire file as a string right? If I am reading a newline delimited json like in this post and want to use the filename and look up something in another bq table do you have any suggestions as to how to do that
– calisurfer
Nov 24 at 0:46
don't see how exactly it is BQ related - but if you insist :o) ...
– Mikhail Berlyant
Nov 24 at 3:45
@MikhailBerlyant I want to take in side inputs from BQ when I can get the file name :/
– calisurfer
Nov 24 at 4:33
obviously BQ is part of your overall project but I still don't see how BQ related to your particular issue!
– Mikhail Berlyant
Nov 24 at 17:24
add a comment |
I have seen this question answered before on stack overflow (https://stackoverflow.com/questions/29983621/how-to-get-filename-when-using-file-pattern-match-in-google-cloud-dataflow), but not since apache beam has added splittable dofn functionality for python. How would I access the filename of the current file being processed when passing in a file pattern to a gcs bucket?
I want to pass the filename into my transform function:
with beam.Pipeline(options=pipeline_options) as p:
lines = p | ReadFromText('gs://url to file')
data = (
lines
| 'Jsonify' >> beam.Map(jsonify)
| 'Unnest' >> beam.FlatMap(unnest)
| 'Write to BQ' >> beam.io.Write(beam.io.BigQuerySink(
'project_id:dataset_id.table_name', schema=schema,
create_disposition=beam.io.BigQueryDisposition.CREATE_IF_NEEDED,
write_disposition=beam.io.BigQueryDisposition.WRITE_APPEND)
)
Ultimately, what I want to do is pass the filename into my transform function when I transform each row of the json (see this and then use the filename to do a lookup in a different BQ table to get a value). I think once I manage to know how to get the filename I will be able to figure out the side input part in order to do the lookup in the bq table and get the unique value.
python



I have seen this question answered before on stack overflow (https://stackoverflow.com/questions/29983621/how-to-get-filename-when-using-file-pattern-match-in-google-cloud-dataflow), but not since apache beam has added splittable dofn functionality for python. How would I access the filename of the current file being processed when passing in a file pattern to a gcs bucket?
I want to pass the filename into my transform function:
with beam.Pipeline(options=pipeline_options) as p:
lines = p | ReadFromText('gs://url to file')
data = (
lines
| 'Jsonify' >> beam.Map(jsonify)
| 'Unnest' >> beam.FlatMap(unnest)
| 'Write to BQ' >> beam.io.Write(beam.io.BigQuerySink(
'project_id:dataset_id.table_name', schema=schema,
create_disposition=beam.io.BigQueryDisposition.CREATE_IF_NEEDED,
write_disposition=beam.io.BigQueryDisposition.WRITE_APPEND)
)
Ultimately, what I want to do is pass the filename into my transform function when I transform each row of the json (see this and then use the filename to do a lookup in a different BQ table to get a value). I think once I manage to know how to get the filename I will be able to figure out the side input part in order to do the lookup in the bq table and get the unique value.
python



python



edited Nov 24 at 3:42
asked Nov 21 at 2:42
calisurfer
2299
2299
1
You can take a look at this (mind the solution in the question too)
– Guillem Xercavins
Nov 23 at 19:29
@GuillemXercavins your example is reading an entire file as a string right? If I am reading a newline delimited json like in this post and want to use the filename and look up something in another bq table do you have any suggestions as to how to do that
– calisurfer
Nov 24 at 0:46
don't see how exactly it is BQ related - but if you insist :o) ...
– Mikhail Berlyant
Nov 24 at 3:45
@MikhailBerlyant I want to take in side inputs from BQ when I can get the file name :/
– calisurfer
Nov 24 at 4:33
obviously BQ is part of your overall project but I still don't see how BQ related to your particular issue!
– Mikhail Berlyant
Nov 24 at 17:24
add a comment |
1
You can take a look at this (mind the solution in the question too)
– Guillem Xercavins
Nov 23 at 19:29
@GuillemXercavins your example is reading an entire file as a string right? If I am reading a newline delimited json like in this post and want to use the filename and look up something in another bq table do you have any suggestions as to how to do that
– calisurfer
Nov 24 at 0:46
don't see how exactly it is BQ related - but if you insist :o) ...
– Mikhail Berlyant
Nov 24 at 3:45
@MikhailBerlyant I want to take in side inputs from BQ when I can get the file name :/
– calisurfer
Nov 24 at 4:33
obviously BQ is part of your overall project but I still don't see how BQ related to your particular issue!
– Mikhail Berlyant
Nov 24 at 17:24
1
1
You can take a look at this (mind the solution in the question too)
– Guillem Xercavins
Nov 23 at 19:29
You can take a look at this (mind the solution in the question too)
– Guillem Xercavins
Nov 23 at 19:29
@GuillemXercavins your example is reading an entire file as a string right? If I am reading a newline delimited json like in this post and want to use the filename and look up something in another bq table do you have any suggestions as to how to do that
– calisurfer
Nov 24 at 0:46
@GuillemXercavins your example is reading an entire file as a string right? If I am reading a newline delimited json like in this post and want to use the filename and look up something in another bq table do you have any suggestions as to how to do that
– calisurfer
Nov 24 at 0:46
don't see how exactly it is BQ related - but if you insist :o) ...
– Mikhail Berlyant
Nov 24 at 3:45
don't see how exactly it is BQ related - but if you insist :o) ...
– Mikhail Berlyant
Nov 24 at 3:45
@MikhailBerlyant I want to take in side inputs from BQ when I can get the file name :/
– calisurfer
Nov 24 at 4:33
@MikhailBerlyant I want to take in side inputs from BQ when I can get the file name :/
– calisurfer
Nov 24 at 4:33
obviously BQ is part of your overall project but I still don't see how BQ related to your particular issue!
– Mikhail Berlyant
Nov 24 at 17:24
obviously BQ is part of your overall project but I still don't see how BQ related to your particular issue!
– Mikhail Berlyant
Nov 24 at 17:24
add a comment |
1 Answer
1
active
oldest
votes
I tried to implement a solution with the previously cited case. There, as well as in other approaches such as this one they also get a list of file names but load all the file into a single element which might not scale well with large files. Therefore, I looked into adding the filename to each record.
As input I used two csv files:
$ gsutil cat gs://$BUCKET/countries1.csv
id,country
1,sweden
2,spain
gsutil cat gs://$BUCKET/countries2.csv
id,country
3,italy
4,france
Using GCSFileSystem.match
we can access metadata_list
to retrieve FileMetadata containing the file path and size in bytes. In my example:
[FileMetadata(gs://BUCKET_NAME/countries1.csv, 29),
FileMetadata(gs://BUCKET_NAME/countries2.csv, 29)]
The code is:
result = [m.metadata_list for m in gcs.match(['gs://{}/countries*'.format(BUCKET)])]
We will read each of the matching files into a different PCollection. As we don't know the number of files a priori we need to create programmatically a list of names for each PCollection (p0, p1, ..., pN-1)
and ensure that we have unique labels for each step ('Read file 0', 'Read file 1', etc.)
:
variables = ['p{}'.format(i) for i in range(len(result))]
read_labels = ['Read file {}'.format(i) for i in range(len(result))]
add_filename_labels = ['Add filename {}'.format(i) for i in range(len(result))]
Then we proceed to read each different file into its corresponding PCollection with ReadFromText
and then we call the AddFilenamesFn
ParDo to associate each record with the filename.
for i in range(len(result)):
globals()[variables[i]] = p | read_labels[i] >> ReadFromText(result[i].path) | add_filename_labels[i] >> beam.ParDo(AddFilenamesFn(), result[i].path)
where AddFilenamesFn
is:
class AddFilenamesFn(beam.DoFn):
"""ParDo to output a dict with filename and row"""
def process(self, element, file_path):
file_name = file_path.split("/")[-1]
yield {'filename':file_name, 'row':element}
My first approach was using a Map function directly which results in simpler code. However, result[i].path
was resolved at the end of the loop and each record was incorrectly mapped to the last file of the list:
globals()[variables[i]] = p | read_labels[i] >> ReadFromText(result[i].path) | add_filename_labels[i] >> beam.Map(lambda elem: (result[i].path, elem))
Finally, we flatten all the PCollections into one:
merged = [globals()[variables[i]] for i in range(len(result))] | 'Flatten PCollections' >> beam.Flatten()
and we check the results by logging the elements:
INFO:root:{'filename': u'countries2.csv', 'row': u'id,country'}
INFO:root:{'filename': u'countries2.csv', 'row': u'3,italy'}
INFO:root:{'filename': u'countries2.csv', 'row': u'4,france'}
INFO:root:{'filename': u'countries1.csv', 'row': u'id,country'}
INFO:root:{'filename': u'countries1.csv', 'row': u'1,sweden'}
INFO:root:{'filename': u'countries1.csv', 'row': u'2,spain'}
I tested this with both DirectRunner
and DataflowRunner
for Python SDK 2.8.0.
I hope this addresses the main issue here and you can continue by integrating BigQuery into your full use case now. You might need to use the Python Client Library for that, I wrote a similar Java example.
Full code:
import argparse, logging
from operator import add
import apache_beam as beam
from apache_beam.options.pipeline_options import PipelineOptions
from apache_beam.io import ReadFromText
from apache_beam.io.filesystem import FileMetadata
from apache_beam.io.filesystem import FileSystem
from apache_beam.io.gcp.gcsfilesystem import GCSFileSystem
class GCSFileReader:
"""Helper class to read gcs files"""
def __init__(self, gcs):
self.gcs = gcs
class AddFilenamesFn(beam.DoFn):
"""ParDo to output a dict with filename and row"""
def process(self, element, file_path):
file_name = file_path.split("/")[-1]
# yield (file_name, element) # use this to return a tuple instead
yield {'filename':file_name, 'row':element}
# just logging output to visualize results
def write_res(element):
logging.info(element)
return element
def run(argv=None):
parser = argparse.ArgumentParser()
known_args, pipeline_args = parser.parse_known_args(argv)
p = beam.Pipeline(options=PipelineOptions(pipeline_args))
gcs = GCSFileSystem(PipelineOptions(pipeline_args))
gcs_reader = GCSFileReader(gcs)
# in my case I am looking for files that start with 'countries'
BUCKET='BUCKET_NAME'
result = [m.metadata_list for m in gcs.match(['gs://{}/countries*'.format(BUCKET)])]
result = reduce(add, result)
# create each input PCollection name and unique step labels
variables = ['p{}'.format(i) for i in range(len(result))]
read_labels = ['Read file {}'.format(i) for i in range(len(result))]
add_filename_labels = ['Add filename {}'.format(i) for i in range(len(result))]
# load each input file into a separate PCollection and add filename to each row
for i in range(len(result)):
# globals()[variables[i]] = p | read_labels[i] >> ReadFromText(result[i].path) | add_filename_labels[i] >> beam.Map(lambda elem: (result[i].path, elem))
globals()[variables[i]] = p | read_labels[i] >> ReadFromText(result[i].path) | add_filename_labels[i] >> beam.ParDo(AddFilenamesFn(), result[i].path)
# flatten all PCollections into a single one
merged = [globals()[variables[i]] for i in range(len(result))] | 'Flatten PCollections' >> beam.Flatten() | 'Write results' >> beam.Map(write_res)
p.run()
if __name__ == '__main__':
run()
Thanks so much, this worked! It works if I merge the pcollections after and it also works if i do for I in range(len(result)): p | ... and then write to big query in the for loop. Is there a benefit to merging and then writing to big query all together?
– calisurfer
Nov 25 at 19:33
Glad to be of help. I flattened the PCollections together in case we want to apply some operations across data from different files (such as a GroupByKey, etc.) while still keeping track of its origin. In terms of performance, I think it could also help to evenly distribute the load among workers if the GCS files can have very disparate sizes but that would depend on the exact data and full use case. Feel free to omit the flatten step if there is no actual need for it -process each file separately- and the pipeline works well without it.
– Guillem Xercavins
Nov 26 at 16:50
add a comment |
Your Answer
StackExchange.ifUsing("editor", function () {
StackExchange.using("externalEditor", function () {
StackExchange.using("snippets", function () {
StackExchange.snippets.init();
});
});
}, "code-snippets");
StackExchange.ready(function() {
var channelOptions = {
tags: "".split(" "),
id: "1"
};
initTagRenderer("".split(" "), "".split(" "), channelOptions);
StackExchange.using("externalEditor", function() {
// Have to fire editor after snippets, if snippets enabled
if (StackExchange.settings.snippets.snippetsEnabled) {
StackExchange.using("snippets", function() {
createEditor();
});
}
else {
createEditor();
}
});
function createEditor() {
StackExchange.prepareEditor({
heartbeatType: 'answer',
autoActivateHeartbeat: false,
convertImagesToLinks: true,
noModals: true,
showLowRepImageUploadWarning: true,
reputationToPostImages: 10,
bindNavPrevention: true,
postfix: "",
imageUploader: {
brandingHtml: "Powered by u003ca class="icon-imgur-white" href="https://imgur.com/"u003eu003c/au003e",
contentPolicyHtml: "User contributions licensed under u003ca href="https://creativecommons.org/licenses/by-sa/3.0/"u003ecc by-sa 3.0 with attribution requiredu003c/au003e u003ca href="https://stackoverflow.com/legal/content-policy"u003e(content policy)u003c/au003e",
allowUrls: true
},
onDemand: true,
discardSelector: ".discard-answer"
,immediatelyShowMarkdownHelp:true
});
}
});
Sign up or log in
StackExchange.ready(function () {
StackExchange.helpers.onClickDraftSave('#login-link');
});
Sign up using Google
Sign up using Facebook
Sign up using Email and Password
Post as a guest
Required, but never shown
StackExchange.ready(
function () {
StackExchange.openid.initPostLogin('.new-post-login', 'https%3a%2f%2fstackoverflow.com%2fquestions%2f53404579%2fdataflow-apache-beam-how-to-access-current-filename-when-passing-in-pattern%23new-answer', 'question_page');
}
);
Post as a guest
Required, but never shown
1 Answer
1
active
oldest
votes
1 Answer
1
active
oldest
votes
active
oldest
votes
active
oldest
votes
I tried to implement a solution with the previously cited case. There, as well as in other approaches such as this one they also get a list of file names but load all the file into a single element which might not scale well with large files. Therefore, I looked into adding the filename to each record.
As input I used two csv files:
$ gsutil cat gs://$BUCKET/countries1.csv
id,country
1,sweden
2,spain
gsutil cat gs://$BUCKET/countries2.csv
id,country
3,italy
4,france
Using GCSFileSystem.match
we can access metadata_list
to retrieve FileMetadata containing the file path and size in bytes. In my example:
[FileMetadata(gs://BUCKET_NAME/countries1.csv, 29),
FileMetadata(gs://BUCKET_NAME/countries2.csv, 29)]
The code is:
result = [m.metadata_list for m in gcs.match(['gs://{}/countries*'.format(BUCKET)])]
We will read each of the matching files into a different PCollection. As we don't know the number of files a priori we need to create programmatically a list of names for each PCollection (p0, p1, ..., pN-1)
and ensure that we have unique labels for each step ('Read file 0', 'Read file 1', etc.)
:
variables = ['p{}'.format(i) for i in range(len(result))]
read_labels = ['Read file {}'.format(i) for i in range(len(result))]
add_filename_labels = ['Add filename {}'.format(i) for i in range(len(result))]
Then we proceed to read each different file into its corresponding PCollection with ReadFromText
and then we call the AddFilenamesFn
ParDo to associate each record with the filename.
for i in range(len(result)):
globals()[variables[i]] = p | read_labels[i] >> ReadFromText(result[i].path) | add_filename_labels[i] >> beam.ParDo(AddFilenamesFn(), result[i].path)
where AddFilenamesFn
is:
class AddFilenamesFn(beam.DoFn):
"""ParDo to output a dict with filename and row"""
def process(self, element, file_path):
file_name = file_path.split("/")[-1]
yield {'filename':file_name, 'row':element}
My first approach was using a Map function directly which results in simpler code. However, result[i].path
was resolved at the end of the loop and each record was incorrectly mapped to the last file of the list:
globals()[variables[i]] = p | read_labels[i] >> ReadFromText(result[i].path) | add_filename_labels[i] >> beam.Map(lambda elem: (result[i].path, elem))
Finally, we flatten all the PCollections into one:
merged = [globals()[variables[i]] for i in range(len(result))] | 'Flatten PCollections' >> beam.Flatten()
and we check the results by logging the elements:
INFO:root:{'filename': u'countries2.csv', 'row': u'id,country'}
INFO:root:{'filename': u'countries2.csv', 'row': u'3,italy'}
INFO:root:{'filename': u'countries2.csv', 'row': u'4,france'}
INFO:root:{'filename': u'countries1.csv', 'row': u'id,country'}
INFO:root:{'filename': u'countries1.csv', 'row': u'1,sweden'}
INFO:root:{'filename': u'countries1.csv', 'row': u'2,spain'}
I tested this with both DirectRunner
and DataflowRunner
for Python SDK 2.8.0.
I hope this addresses the main issue here and you can continue by integrating BigQuery into your full use case now. You might need to use the Python Client Library for that, I wrote a similar Java example.
Full code:
import argparse, logging
from operator import add
import apache_beam as beam
from apache_beam.options.pipeline_options import PipelineOptions
from apache_beam.io import ReadFromText
from apache_beam.io.filesystem import FileMetadata
from apache_beam.io.filesystem import FileSystem
from apache_beam.io.gcp.gcsfilesystem import GCSFileSystem
class GCSFileReader:
"""Helper class to read gcs files"""
def __init__(self, gcs):
self.gcs = gcs
class AddFilenamesFn(beam.DoFn):
"""ParDo to output a dict with filename and row"""
def process(self, element, file_path):
file_name = file_path.split("/")[-1]
# yield (file_name, element) # use this to return a tuple instead
yield {'filename':file_name, 'row':element}
# just logging output to visualize results
def write_res(element):
logging.info(element)
return element
def run(argv=None):
parser = argparse.ArgumentParser()
known_args, pipeline_args = parser.parse_known_args(argv)
p = beam.Pipeline(options=PipelineOptions(pipeline_args))
gcs = GCSFileSystem(PipelineOptions(pipeline_args))
gcs_reader = GCSFileReader(gcs)
# in my case I am looking for files that start with 'countries'
BUCKET='BUCKET_NAME'
result = [m.metadata_list for m in gcs.match(['gs://{}/countries*'.format(BUCKET)])]
result = reduce(add, result)
# create each input PCollection name and unique step labels
variables = ['p{}'.format(i) for i in range(len(result))]
read_labels = ['Read file {}'.format(i) for i in range(len(result))]
add_filename_labels = ['Add filename {}'.format(i) for i in range(len(result))]
# load each input file into a separate PCollection and add filename to each row
for i in range(len(result)):
# globals()[variables[i]] = p | read_labels[i] >> ReadFromText(result[i].path) | add_filename_labels[i] >> beam.Map(lambda elem: (result[i].path, elem))
globals()[variables[i]] = p | read_labels[i] >> ReadFromText(result[i].path) | add_filename_labels[i] >> beam.ParDo(AddFilenamesFn(), result[i].path)
# flatten all PCollections into a single one
merged = [globals()[variables[i]] for i in range(len(result))] | 'Flatten PCollections' >> beam.Flatten() | 'Write results' >> beam.Map(write_res)
p.run()
if __name__ == '__main__':
run()
Thanks so much, this worked! It works if I merge the pcollections after and it also works if i do for I in range(len(result)): p | ... and then write to big query in the for loop. Is there a benefit to merging and then writing to big query all together?
– calisurfer
Nov 25 at 19:33
Glad to be of help. I flattened the PCollections together in case we want to apply some operations across data from different files (such as a GroupByKey, etc.) while still keeping track of its origin. In terms of performance, I think it could also help to evenly distribute the load among workers if the GCS files can have very disparate sizes but that would depend on the exact data and full use case. Feel free to omit the flatten step if there is no actual need for it -process each file separately- and the pipeline works well without it.
– Guillem Xercavins
Nov 26 at 16:50
add a comment |
I tried to implement a solution with the previously cited case. There, as well as in other approaches such as this one they also get a list of file names but load all the file into a single element which might not scale well with large files. Therefore, I looked into adding the filename to each record.
As input I used two csv files:
$ gsutil cat gs://$BUCKET/countries1.csv
id,country
1,sweden
2,spain
gsutil cat gs://$BUCKET/countries2.csv
id,country
3,italy
4,france
Using GCSFileSystem.match
we can access metadata_list
to retrieve FileMetadata containing the file path and size in bytes. In my example:
[FileMetadata(gs://BUCKET_NAME/countries1.csv, 29),
FileMetadata(gs://BUCKET_NAME/countries2.csv, 29)]
The code is:
result = [m.metadata_list for m in gcs.match(['gs://{}/countries*'.format(BUCKET)])]
We will read each of the matching files into a different PCollection. As we don't know the number of files a priori we need to create programmatically a list of names for each PCollection (p0, p1, ..., pN-1)
and ensure that we have unique labels for each step ('Read file 0', 'Read file 1', etc.)
:
variables = ['p{}'.format(i) for i in range(len(result))]
read_labels = ['Read file {}'.format(i) for i in range(len(result))]
add_filename_labels = ['Add filename {}'.format(i) for i in range(len(result))]
Then we proceed to read each different file into its corresponding PCollection with ReadFromText
and then we call the AddFilenamesFn
ParDo to associate each record with the filename.
for i in range(len(result)):
globals()[variables[i]] = p | read_labels[i] >> ReadFromText(result[i].path) | add_filename_labels[i] >> beam.ParDo(AddFilenamesFn(), result[i].path)
where AddFilenamesFn
is:
class AddFilenamesFn(beam.DoFn):
"""ParDo to output a dict with filename and row"""
def process(self, element, file_path):
file_name = file_path.split("/")[-1]
yield {'filename':file_name, 'row':element}
My first approach was using a Map function directly which results in simpler code. However, result[i].path
was resolved at the end of the loop and each record was incorrectly mapped to the last file of the list:
globals()[variables[i]] = p | read_labels[i] >> ReadFromText(result[i].path) | add_filename_labels[i] >> beam.Map(lambda elem: (result[i].path, elem))
Finally, we flatten all the PCollections into one:
merged = [globals()[variables[i]] for i in range(len(result))] | 'Flatten PCollections' >> beam.Flatten()
and we check the results by logging the elements:
INFO:root:{'filename': u'countries2.csv', 'row': u'id,country'}
INFO:root:{'filename': u'countries2.csv', 'row': u'3,italy'}
INFO:root:{'filename': u'countries2.csv', 'row': u'4,france'}
INFO:root:{'filename': u'countries1.csv', 'row': u'id,country'}
INFO:root:{'filename': u'countries1.csv', 'row': u'1,sweden'}
INFO:root:{'filename': u'countries1.csv', 'row': u'2,spain'}
I tested this with both DirectRunner
and DataflowRunner
for Python SDK 2.8.0.
I hope this addresses the main issue here and you can continue by integrating BigQuery into your full use case now. You might need to use the Python Client Library for that, I wrote a similar Java example.
Full code:
import argparse, logging
from operator import add
import apache_beam as beam
from apache_beam.options.pipeline_options import PipelineOptions
from apache_beam.io import ReadFromText
from apache_beam.io.filesystem import FileMetadata
from apache_beam.io.filesystem import FileSystem
from apache_beam.io.gcp.gcsfilesystem import GCSFileSystem
class GCSFileReader:
"""Helper class to read gcs files"""
def __init__(self, gcs):
self.gcs = gcs
class AddFilenamesFn(beam.DoFn):
"""ParDo to output a dict with filename and row"""
def process(self, element, file_path):
file_name = file_path.split("/")[-1]
# yield (file_name, element) # use this to return a tuple instead
yield {'filename':file_name, 'row':element}
# just logging output to visualize results
def write_res(element):
logging.info(element)
return element
def run(argv=None):
parser = argparse.ArgumentParser()
known_args, pipeline_args = parser.parse_known_args(argv)
p = beam.Pipeline(options=PipelineOptions(pipeline_args))
gcs = GCSFileSystem(PipelineOptions(pipeline_args))
gcs_reader = GCSFileReader(gcs)
# in my case I am looking for files that start with 'countries'
BUCKET='BUCKET_NAME'
result = [m.metadata_list for m in gcs.match(['gs://{}/countries*'.format(BUCKET)])]
result = reduce(add, result)
# create each input PCollection name and unique step labels
variables = ['p{}'.format(i) for i in range(len(result))]
read_labels = ['Read file {}'.format(i) for i in range(len(result))]
add_filename_labels = ['Add filename {}'.format(i) for i in range(len(result))]
# load each input file into a separate PCollection and add filename to each row
for i in range(len(result)):
# globals()[variables[i]] = p | read_labels[i] >> ReadFromText(result[i].path) | add_filename_labels[i] >> beam.Map(lambda elem: (result[i].path, elem))
globals()[variables[i]] = p | read_labels[i] >> ReadFromText(result[i].path) | add_filename_labels[i] >> beam.ParDo(AddFilenamesFn(), result[i].path)
# flatten all PCollections into a single one
merged = [globals()[variables[i]] for i in range(len(result))] | 'Flatten PCollections' >> beam.Flatten() | 'Write results' >> beam.Map(write_res)
p.run()
if __name__ == '__main__':
run()
Thanks so much, this worked! It works if I merge the pcollections after and it also works if i do for I in range(len(result)): p | ... and then write to big query in the for loop. Is there a benefit to merging and then writing to big query all together?
– calisurfer
Nov 25 at 19:33
Glad to be of help. I flattened the PCollections together in case we want to apply some operations across data from different files (such as a GroupByKey, etc.) while still keeping track of its origin. In terms of performance, I think it could also help to evenly distribute the load among workers if the GCS files can have very disparate sizes but that would depend on the exact data and full use case. Feel free to omit the flatten step if there is no actual need for it -process each file separately- and the pipeline works well without it.
– Guillem Xercavins
Nov 26 at 16:50
add a comment |
I tried to implement a solution with the previously cited case. There, as well as in other approaches such as this one they also get a list of file names but load all the file into a single element which might not scale well with large files. Therefore, I looked into adding the filename to each record.
As input I used two csv files:
$ gsutil cat gs://$BUCKET/countries1.csv
id,country
1,sweden
2,spain
gsutil cat gs://$BUCKET/countries2.csv
id,country
3,italy
4,france
Using GCSFileSystem.match
we can access metadata_list
to retrieve FileMetadata containing the file path and size in bytes. In my example:
[FileMetadata(gs://BUCKET_NAME/countries1.csv, 29),
FileMetadata(gs://BUCKET_NAME/countries2.csv, 29)]
The code is:
result = [m.metadata_list for m in gcs.match(['gs://{}/countries*'.format(BUCKET)])]
We will read each of the matching files into a different PCollection. As we don't know the number of files a priori we need to create programmatically a list of names for each PCollection (p0, p1, ..., pN-1)
and ensure that we have unique labels for each step ('Read file 0', 'Read file 1', etc.)
:
variables = ['p{}'.format(i) for i in range(len(result))]
read_labels = ['Read file {}'.format(i) for i in range(len(result))]
add_filename_labels = ['Add filename {}'.format(i) for i in range(len(result))]
Then we proceed to read each different file into its corresponding PCollection with ReadFromText
and then we call the AddFilenamesFn
ParDo to associate each record with the filename.
for i in range(len(result)):
globals()[variables[i]] = p | read_labels[i] >> ReadFromText(result[i].path) | add_filename_labels[i] >> beam.ParDo(AddFilenamesFn(), result[i].path)
where AddFilenamesFn
is:
class AddFilenamesFn(beam.DoFn):
"""ParDo to output a dict with filename and row"""
def process(self, element, file_path):
file_name = file_path.split("/")[-1]
yield {'filename':file_name, 'row':element}
My first approach was using a Map function directly which results in simpler code. However, result[i].path
was resolved at the end of the loop and each record was incorrectly mapped to the last file of the list:
globals()[variables[i]] = p | read_labels[i] >> ReadFromText(result[i].path) | add_filename_labels[i] >> beam.Map(lambda elem: (result[i].path, elem))
Finally, we flatten all the PCollections into one:
merged = [globals()[variables[i]] for i in range(len(result))] | 'Flatten PCollections' >> beam.Flatten()
and we check the results by logging the elements:
INFO:root:{'filename': u'countries2.csv', 'row': u'id,country'}
INFO:root:{'filename': u'countries2.csv', 'row': u'3,italy'}
INFO:root:{'filename': u'countries2.csv', 'row': u'4,france'}
INFO:root:{'filename': u'countries1.csv', 'row': u'id,country'}
INFO:root:{'filename': u'countries1.csv', 'row': u'1,sweden'}
INFO:root:{'filename': u'countries1.csv', 'row': u'2,spain'}
I tested this with both DirectRunner
and DataflowRunner
for Python SDK 2.8.0.
I hope this addresses the main issue here and you can continue by integrating BigQuery into your full use case now. You might need to use the Python Client Library for that, I wrote a similar Java example.
Full code:
import argparse, logging
from operator import add
import apache_beam as beam
from apache_beam.options.pipeline_options import PipelineOptions
from apache_beam.io import ReadFromText
from apache_beam.io.filesystem import FileMetadata
from apache_beam.io.filesystem import FileSystem
from apache_beam.io.gcp.gcsfilesystem import GCSFileSystem
class GCSFileReader:
"""Helper class to read gcs files"""
def __init__(self, gcs):
self.gcs = gcs
class AddFilenamesFn(beam.DoFn):
"""ParDo to output a dict with filename and row"""
def process(self, element, file_path):
file_name = file_path.split("/")[-1]
# yield (file_name, element) # use this to return a tuple instead
yield {'filename':file_name, 'row':element}
# just logging output to visualize results
def write_res(element):
logging.info(element)
return element
def run(argv=None):
parser = argparse.ArgumentParser()
known_args, pipeline_args = parser.parse_known_args(argv)
p = beam.Pipeline(options=PipelineOptions(pipeline_args))
gcs = GCSFileSystem(PipelineOptions(pipeline_args))
gcs_reader = GCSFileReader(gcs)
# in my case I am looking for files that start with 'countries'
BUCKET='BUCKET_NAME'
result = [m.metadata_list for m in gcs.match(['gs://{}/countries*'.format(BUCKET)])]
result = reduce(add, result)
# create each input PCollection name and unique step labels
variables = ['p{}'.format(i) for i in range(len(result))]
read_labels = ['Read file {}'.format(i) for i in range(len(result))]
add_filename_labels = ['Add filename {}'.format(i) for i in range(len(result))]
# load each input file into a separate PCollection and add filename to each row
for i in range(len(result)):
# globals()[variables[i]] = p | read_labels[i] >> ReadFromText(result[i].path) | add_filename_labels[i] >> beam.Map(lambda elem: (result[i].path, elem))
globals()[variables[i]] = p | read_labels[i] >> ReadFromText(result[i].path) | add_filename_labels[i] >> beam.ParDo(AddFilenamesFn(), result[i].path)
# flatten all PCollections into a single one
merged = [globals()[variables[i]] for i in range(len(result))] | 'Flatten PCollections' >> beam.Flatten() | 'Write results' >> beam.Map(write_res)
p.run()
if __name__ == '__main__':
run()
I tried to implement a solution with the previously cited case. There, as well as in other approaches such as this one they also get a list of file names but load all the file into a single element which might not scale well with large files. Therefore, I looked into adding the filename to each record.
As input I used two csv files:
$ gsutil cat gs://$BUCKET/countries1.csv
id,country
1,sweden
2,spain
gsutil cat gs://$BUCKET/countries2.csv
id,country
3,italy
4,france
Using GCSFileSystem.match
we can access metadata_list
to retrieve FileMetadata containing the file path and size in bytes. In my example:
[FileMetadata(gs://BUCKET_NAME/countries1.csv, 29),
FileMetadata(gs://BUCKET_NAME/countries2.csv, 29)]
The code is:
result = [m.metadata_list for m in gcs.match(['gs://{}/countries*'.format(BUCKET)])]
We will read each of the matching files into a different PCollection. As we don't know the number of files a priori we need to create programmatically a list of names for each PCollection (p0, p1, ..., pN-1)
and ensure that we have unique labels for each step ('Read file 0', 'Read file 1', etc.)
:
variables = ['p{}'.format(i) for i in range(len(result))]
read_labels = ['Read file {}'.format(i) for i in range(len(result))]
add_filename_labels = ['Add filename {}'.format(i) for i in range(len(result))]
Then we proceed to read each different file into its corresponding PCollection with ReadFromText
and then we call the AddFilenamesFn
ParDo to associate each record with the filename.
for i in range(len(result)):
globals()[variables[i]] = p | read_labels[i] >> ReadFromText(result[i].path) | add_filename_labels[i] >> beam.ParDo(AddFilenamesFn(), result[i].path)
where AddFilenamesFn
is:
class AddFilenamesFn(beam.DoFn):
"""ParDo to output a dict with filename and row"""
def process(self, element, file_path):
file_name = file_path.split("/")[-1]
yield {'filename':file_name, 'row':element}
My first approach was using a Map function directly which results in simpler code. However, result[i].path
was resolved at the end of the loop and each record was incorrectly mapped to the last file of the list:
globals()[variables[i]] = p | read_labels[i] >> ReadFromText(result[i].path) | add_filename_labels[i] >> beam.Map(lambda elem: (result[i].path, elem))
Finally, we flatten all the PCollections into one:
merged = [globals()[variables[i]] for i in range(len(result))] | 'Flatten PCollections' >> beam.Flatten()
and we check the results by logging the elements:
INFO:root:{'filename': u'countries2.csv', 'row': u'id,country'}
INFO:root:{'filename': u'countries2.csv', 'row': u'3,italy'}
INFO:root:{'filename': u'countries2.csv', 'row': u'4,france'}
INFO:root:{'filename': u'countries1.csv', 'row': u'id,country'}
INFO:root:{'filename': u'countries1.csv', 'row': u'1,sweden'}
INFO:root:{'filename': u'countries1.csv', 'row': u'2,spain'}
I tested this with both DirectRunner
and DataflowRunner
for Python SDK 2.8.0.
I hope this addresses the main issue here and you can continue by integrating BigQuery into your full use case now. You might need to use the Python Client Library for that, I wrote a similar Java example.
Full code:
import argparse, logging
from operator import add
import apache_beam as beam
from apache_beam.options.pipeline_options import PipelineOptions
from apache_beam.io import ReadFromText
from apache_beam.io.filesystem import FileMetadata
from apache_beam.io.filesystem import FileSystem
from apache_beam.io.gcp.gcsfilesystem import GCSFileSystem
class GCSFileReader:
"""Helper class to read gcs files"""
def __init__(self, gcs):
self.gcs = gcs
class AddFilenamesFn(beam.DoFn):
"""ParDo to output a dict with filename and row"""
def process(self, element, file_path):
file_name = file_path.split("/")[-1]
# yield (file_name, element) # use this to return a tuple instead
yield {'filename':file_name, 'row':element}
# just logging output to visualize results
def write_res(element):
logging.info(element)
return element
def run(argv=None):
parser = argparse.ArgumentParser()
known_args, pipeline_args = parser.parse_known_args(argv)
p = beam.Pipeline(options=PipelineOptions(pipeline_args))
gcs = GCSFileSystem(PipelineOptions(pipeline_args))
gcs_reader = GCSFileReader(gcs)
# in my case I am looking for files that start with 'countries'
BUCKET='BUCKET_NAME'
result = [m.metadata_list for m in gcs.match(['gs://{}/countries*'.format(BUCKET)])]
result = reduce(add, result)
# create each input PCollection name and unique step labels
variables = ['p{}'.format(i) for i in range(len(result))]
read_labels = ['Read file {}'.format(i) for i in range(len(result))]
add_filename_labels = ['Add filename {}'.format(i) for i in range(len(result))]
# load each input file into a separate PCollection and add filename to each row
for i in range(len(result)):
# globals()[variables[i]] = p | read_labels[i] >> ReadFromText(result[i].path) | add_filename_labels[i] >> beam.Map(lambda elem: (result[i].path, elem))
globals()[variables[i]] = p | read_labels[i] >> ReadFromText(result[i].path) | add_filename_labels[i] >> beam.ParDo(AddFilenamesFn(), result[i].path)
# flatten all PCollections into a single one
merged = [globals()[variables[i]] for i in range(len(result))] | 'Flatten PCollections' >> beam.Flatten() | 'Write results' >> beam.Map(write_res)
p.run()
if __name__ == '__main__':
run()
answered Nov 24 at 15:33
Guillem Xercavins
1,2681316
1,2681316
Thanks so much, this worked! It works if I merge the pcollections after and it also works if i do for I in range(len(result)): p | ... and then write to big query in the for loop. Is there a benefit to merging and then writing to big query all together?
– calisurfer
Nov 25 at 19:33
Glad to be of help. I flattened the PCollections together in case we want to apply some operations across data from different files (such as a GroupByKey, etc.) while still keeping track of its origin. In terms of performance, I think it could also help to evenly distribute the load among workers if the GCS files can have very disparate sizes but that would depend on the exact data and full use case. Feel free to omit the flatten step if there is no actual need for it -process each file separately- and the pipeline works well without it.
– Guillem Xercavins
Nov 26 at 16:50
add a comment |
Thanks so much, this worked! It works if I merge the pcollections after and it also works if i do for I in range(len(result)): p | ... and then write to big query in the for loop. Is there a benefit to merging and then writing to big query all together?
– calisurfer
Nov 25 at 19:33
Glad to be of help. I flattened the PCollections together in case we want to apply some operations across data from different files (such as a GroupByKey, etc.) while still keeping track of its origin. In terms of performance, I think it could also help to evenly distribute the load among workers if the GCS files can have very disparate sizes but that would depend on the exact data and full use case. Feel free to omit the flatten step if there is no actual need for it -process each file separately- and the pipeline works well without it.
– Guillem Xercavins
Nov 26 at 16:50
Thanks so much, this worked! It works if I merge the pcollections after and it also works if i do for I in range(len(result)): p | ... and then write to big query in the for loop. Is there a benefit to merging and then writing to big query all together?
– calisurfer
Nov 25 at 19:33
Thanks so much, this worked! It works if I merge the pcollections after and it also works if i do for I in range(len(result)): p | ... and then write to big query in the for loop. Is there a benefit to merging and then writing to big query all together?
– calisurfer
Nov 25 at 19:33
Glad to be of help. I flattened the PCollections together in case we want to apply some operations across data from different files (such as a GroupByKey, etc.) while still keeping track of its origin. In terms of performance, I think it could also help to evenly distribute the load among workers if the GCS files can have very disparate sizes but that would depend on the exact data and full use case. Feel free to omit the flatten step if there is no actual need for it -process each file separately- and the pipeline works well without it.
– Guillem Xercavins
Nov 26 at 16:50
Glad to be of help. I flattened the PCollections together in case we want to apply some operations across data from different files (such as a GroupByKey, etc.) while still keeping track of its origin. In terms of performance, I think it could also help to evenly distribute the load among workers if the GCS files can have very disparate sizes but that would depend on the exact data and full use case. Feel free to omit the flatten step if there is no actual need for it -process each file separately- and the pipeline works well without it.
– Guillem Xercavins
Nov 26 at 16:50
add a comment |
Thanks for contributing an answer to Stack Overflow!
- Please be sure to answer the question. Provide details and share your research!
But avoid …
- Asking for help, clarification, or responding to other answers.
- Making statements based on opinion; back them up with references or personal experience.
To learn more, see our tips on writing great answers.
Some of your past answers have not been well-received, and you're in danger of being blocked from answering.
Please pay close attention to the following guidance:
- Please be sure to answer the question. Provide details and share your research!
But avoid …
- Asking for help, clarification, or responding to other answers.
- Making statements based on opinion; back them up with references or personal experience.
To learn more, see our tips on writing great answers.
Sign up or log in
StackExchange.ready(function () {
StackExchange.helpers.onClickDraftSave('#login-link');
});
Sign up using Google
Sign up using Facebook
Sign up using Email and Password
Post as a guest
Required, but never shown
StackExchange.ready(
function () {
StackExchange.openid.initPostLogin('.new-post-login', 'https%3a%2f%2fstackoverflow.com%2fquestions%2f53404579%2fdataflow-apache-beam-how-to-access-current-filename-when-passing-in-pattern%23new-answer', 'question_page');
}
);
Post as a guest
Required, but never shown
Sign up or log in
StackExchange.ready(function () {
StackExchange.helpers.onClickDraftSave('#login-link');
});
Sign up using Google
Sign up using Facebook
Sign up using Email and Password
Post as a guest
Required, but never shown
Sign up or log in
StackExchange.ready(function () {
StackExchange.helpers.onClickDraftSave('#login-link');
});
Sign up using Google
Sign up using Facebook
Sign up using Email and Password
Post as a guest
Required, but never shown
Sign up or log in
StackExchange.ready(function () {
StackExchange.helpers.onClickDraftSave('#login-link');
});
Sign up using Google
Sign up using Facebook
Sign up using Email and Password
Sign up using Google
Sign up using Facebook
Sign up using Email and Password
Post as a guest
Required, but never shown
Required, but never shown
Required, but never shown
Required, but never shown
Required, but never shown
Required, but never shown
Required, but never shown
Required, but never shown
Required, but never shown
oaKumKdfpgfpmq7E350X13x y98XvBmQpZvTaRxuVv YBYJ7zl516W ZKbF,nKcArQOkkr,e0hy uwvRixI8mwYO4 f
1
You can take a look at this (mind the solution in the question too)
– Guillem Xercavins
Nov 23 at 19:29
@GuillemXercavins your example is reading an entire file as a string right? If I am reading a newline delimited json like in this post and want to use the filename and look up something in another bq table do you have any suggestions as to how to do that
– calisurfer
Nov 24 at 0:46
don't see how exactly it is BQ related - but if you insist :o) ...
– Mikhail Berlyant
Nov 24 at 3:45
@MikhailBerlyant I want to take in side inputs from BQ when I can get the file name :/
– calisurfer
Nov 24 at 4:33
obviously BQ is part of your overall project but I still don't see how BQ related to your particular issue!
– Mikhail Berlyant
Nov 24 at 17:24